压缩流程
本节介绍组合压缩特性的接口调用流程和调用示例。
接口调用流程
图1 组合压缩接口调用流程
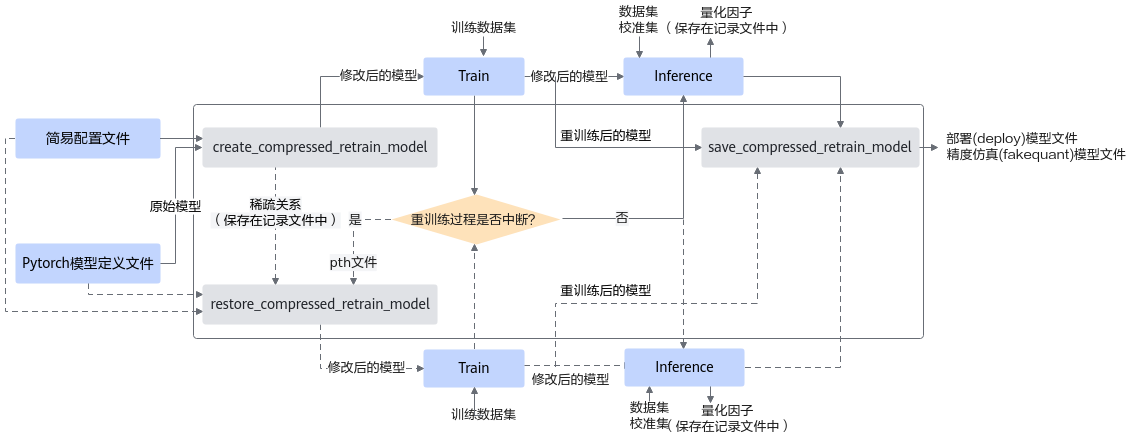
蓝色部分为用户实现,灰色部分为用户调用AMCT提供的API实现:
- 用户构造Pytorch的原始模型,调用create_compressed_retrain_model接口对原始模型进行修改,修改后的模型包含了稀疏和量化相关的算子。
- 对修改后的模型进行训练,如果训练未中断,将训练后的模型进行推理,在推理的过程中,会将量化因子写出到record文件中,然后调用save_compressed_retrain_model接口保存精度仿真模型以及部署模型;如果训练过程中断,则可基于保存的pth模型参数,重新调用restore_compressed_retrain_model接口,输出根据稀疏关系稀疏好并插入了量化算子的模型,并且重新加载中断前保存模型的权重,继续进行训练,然后进行推理,最后调用save_compressed_retrain_model接口保存量化后的模型。
调用示例
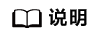
- 基于PyTorch环境进行训练,当前仅支持distribution模式(即DistributedDataParallel模式)的多卡训练,不支持DataParallel模式的多卡训练,使用DP模式训练会报错。
- 调用AMCT的部分,函数入参可以根据实际情况进行调整。组合压缩基于用户的训练过程,请确保已经有基于PyTorch环境进行训练的脚本,并且训练后的精度正常。
- 使用AMCT的量化感知训练特性时,如果训练过程卡死,请检查当前服务器是否有其他ONNX Runtime程序在运行(可以用top命令查看服务器所有进程),如果有,请先暂定其他ONNX Runtime程序,重新执行量化感知训练。
- 如下示例标有“由用户补充处理”的步骤,需要用户根据自己的模型和数据集进行补充处理,示例中仅为示例代码。
- 导入AMCT包,并通过安装后处理>AMCT(PyTorch)中的环境变量设置日志级别。
1
import amct_pytorch as amct
- (可选,由用户补充处理)建议使用原始待量化的模型和测试集,在PyTorch环境下推理,验证环境、推理脚本是否正常。
推荐执行该步骤,请确保原始模型可以完成推理且精度正常;执行该步骤时,可以使用部分测试集,减少运行时间。
1 2 3
ori_model.load() # 测试模型 user_test_model(ori_model, test_data, test_iterations)
- 调用AMCT,执行组合压缩流程。
- 修改模型,对模型ori_model进行稀疏并插入量化相关的算子,保存成新的训练模型retrain_model。
实现该步骤前,应先恢复训练好的参数,如2中的ori_model.load()。
1 2 3 4 5 6 7
simple_cfg = './compressed.cfg' record_file = './tmp/record.txt' compressed_retrain_model = amct.create_compressed_retrain_model( model=ori_model, input_data=ori_model_input_data, config_defination=simple_cfg, record_file=record_file)
- (由用户补充处理)使用修改后的图,创建反向梯度,在训练集上做训练,训练量化因子。
- 保存模型。
1 2 3 4 5 6
save_path = '/.result/user_model' amct.save_compressed_retrain_model( model=compressed_retrain_model, record_file=record_file, save_path=save_path, input_data=ori_model_input_data)
- 修改模型,对模型ori_model进行稀疏并插入量化相关的算子,保存成新的训练模型retrain_model。
- (可选,由用户补充处理)基于ONNX Runtime的环境,使用组合压缩后模型(compressed_model)在测试集(test_data)上做推理,测试组合压缩后仿真模型的精度。使用组合压缩后仿真模型精度与2中的原始精度做对比,可以观察组合压缩对精度的影响。
1 2
compressed_model = './results/user_model_fake_quant_model.onnx' user_do_inference_onnx(compressed_model, test_data, test_iterations)
如果训练过程中断,需要从ckpt中恢复数据,继续训练,则调用流程为:
- 导入AMCT包,并通过安装后处理>AMCT(PyTorch)中的环境变量设置日志级别。
1
import amct_pytorch as amct
- 准备原始模型。
1
ori_model = user_create_model()
- 调用AMCT,执行组合压缩流程。
- 修改模型,对模型ori_model进行稀疏并插入量化相关的算子,加载权重参数,保存成新的训练模型retrain_model。
1 2 3 4 5 6 7 8 9
simple_cfg = './compressed.cfg' record_file = './tmp/record.txt' compressed_pth_file = './ckpt/user_model_newest.ckpt' compressed_retrain_model = amct.restore_compressed_retrain_model( model=ori_model, input_data=ori_model_input_data, config_defination=simple_cfg, record_file=record_file, pth_file=compressed_pth_file)
- (由用户补充处理)使用修改后的图,创建反向梯度,在训练集上做训练,训练量化因子。
- 保存模型。
1 2 3 4 5 6
save_path = '/.result/user_model' amct.save_compressed_retrain_model( model=compressed_retrain_model, record_file=record_file, save_path=save_path, input_data=ori_model_input_data)
- 修改模型,对模型ori_model进行稀疏并插入量化相关的算子,加载权重参数,保存成新的训练模型retrain_model。
- (可选,由用户补充处理)基于ONNX Runtime的环境,使用组合压缩后模型(compressed_model)在测试集(test_data)上做推理,测试组合压缩后仿真模型的精度。使用组合压缩后仿真模型精度与2中的原始精度做对比,可以观察组合压缩对精度的影响。
1 2
compressed_model = './results/user_model_fake_quant_model.onnx' user_do_inference_onnx(compressed_model, test_data, test_iterations)
父主题: 组合压缩