通过FP Buffer存放量化参数实现高效随路量化
【优先级】高
【描述】算子实现中对矩阵乘结果进行量化计算时,可将量化参数搬运到C2PIPE2GM(Fixpipe Buffer)上,调用一次Fixpipe接口实现矩阵乘结果的量化计算。相比于将矩阵乘的结果从CO1(L0C)搬运到GM,再从GM搬运到UB,在UB进行量化计算的过程,数据搬运的次数更少,内存使用效率更高。
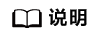
本性能优化手段仅针对Atlas A2训练系列产品/Atlas 800I A2推理产品生效。
图1 反例数据流图
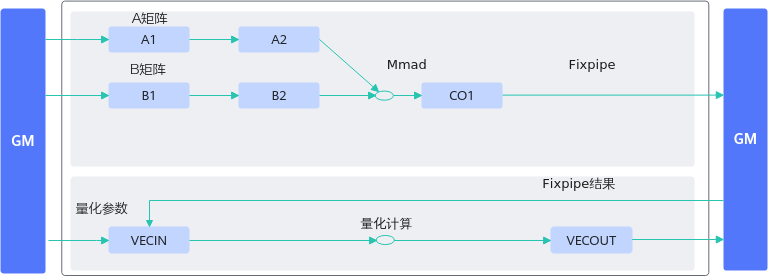
图2 正例数据流图
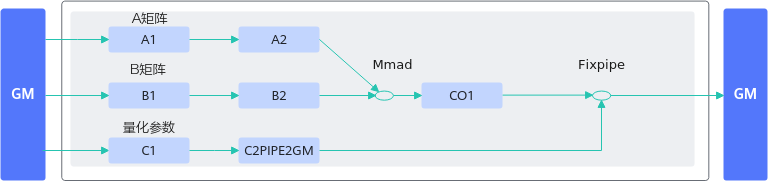
【反例】
对矩阵乘结果进行量化计算的过程如下:
- 将矩阵乘的结果从CO1搬运到workspace上;
- 再从workspace搬运到UB上;
- 将量化参数搬运到UB上,和矩阵乘的结果一起在UB上进行一系列量化计算;
- 将最终量化结果从UB搬运到GM上。
相比于正确示例多增加了CO1->workspace、workspace->UB的搬运过程和量化的vector计算。
... // 该样例仅做示例说明,非完整代码,省略了部分同步控制代码 public: __aicore__ inline KernelSample() { aSize = m * k; bSize = k * n; cSize = m * n; } __aicore__ inline void Init(__gm__ uint8_t *a, __gm__ uint8_t *b, __gm__ uint8_t *c, __gm__ uint8_t *deqTensor) { aGM.SetGlobalBuffer((__gm__ half *)a); bGM.SetGlobalBuffer((__gm__ half *)b); cGM.SetGlobalBuffer((__gm__ float *)c); deqGM.SetGlobalBuffer((__gm__ half *)deqTensor); pipe.InitBuffer(inQueueA1, 1, aSize * sizeof(half)); pipe.InitBuffer(inQueueA2, 1, aSize * sizeof(half)); pipe.InitBuffer(inQueueB1, 1, bSize * sizeof(half)); pipe.InitBuffer(inQueueB2, 2, bSize * sizeof(half)); pipe.InitBuffer(outQueueCO1, 1, cSize * sizeof(float)); pipe.InitBuffer(inQueueSrc0, 1, cSize * sizeof(float)); pipe.InitBuffer(inQueueTmp, 1, cSize * sizeof(half)); pipe.InitBuffer(inQueueDeq, 1, cSize * sizeof(half)); pipe.InitBuffer(outQueueDst, 1, cSize * sizeof(int8_t)); } __aicore__ inline void Process() { CopyIn(); SplitA(); SplitB(); Compute(); CopyOut(); CopyIn1(); Compute1(); CopyOut1(); } private: __aicore__ inline void CopyIn() { LocalTensor<half> a1Local = inQueueA1.AllocTensor<half>(); LocalTensor<half> b1Local = inQueueB1.AllocTensor<half>(); LocalTensor<half> deqLocal = inQueueDeq.AllocTensor<half>(); Nd2NzParams dataCopyA1Params; dataCopyA1Params.ndNum = 1; dataCopyA1Params.nValue = m; dataCopyA1Params.dValue = k; dataCopyA1Params.srcNdMatrixStride = 0; dataCopyA1Params.srcDValue = k; dataCopyA1Params.dstNzC0Stride = m; dataCopyA1Params.dstNzNStride = 1; dataCopyA1Params.dstNzMatrixStride = 0; DataCopy(a1Local, aGM, dataCopyA1Params); Nd2NzParams dataCopyB1Params; dataCopyB1Params.ndNum = 1; dataCopyB1Params.nValue = k; dataCopyB1Params.dValue = n; dataCopyB1Params.srcNdMatrixStride = 0; dataCopyB1Params.srcDValue = n; dataCopyB1Params.dstNzC0Stride = k; dataCopyB1Params.dstNzNStride = 1; dataCopyB1Params.dstNzMatrixStride = 0; DataCopy(b1Local, bGM, dataCopyB1Params); // 将量化参数搬运到UB DataCopy(deqLocal, deqGM, cSize); inQueueA1.EnQue(a1Local); inQueueB1.EnQue(b1Local); inQueueDeq.EnQue(deqLocal); } __aicore__ inline void SplitA() { ... } __aicore__ inline void SplitB() { ... } __aicore__ inline void Compute() { LocalTensor<half> a2Local = inQueueA2.DeQue<half>(); LocalTensor<half> b2Local = inQueueB2.DeQue<half>(); LocalTensor<float> c1Local = outQueueCO1.AllocTensor<float>(); MmadParams mmadParams; mmadParams.m = m; mmadParams.n = n; mmadParams.k = k; // 矩阵乘 Mmad(c1Local, a2Local, b2Local, mmadParams); // m*n outQueueCO1.EnQue<float>(c1Local); inQueueA2.FreeTensor(a2Local); inQueueB2.FreeTensor(b2Local); } __aicore__ inline void CopyOut() { LocalTensor<float> c1Local = outQueueCO1.DeQue<float>(); GM_ADDR usrWorkspace = AscendC::GetUserWorkspace(workspace); xGm.SetGlobalBuffer((__gm__ float *)(usrWorkspace)); FixpipeParamsV220 fixpipeParams; fixpipeParams.nSize = n; fixpipeParams.mSize = m; fixpipeParams.srcStride = m; fixpipeParams.dstStride = n; fixpipeParams.ndNum = 1; fixpipeParams.srcNdStride = 0; fixpipeParams.dstNdStride = 0; // 将矩阵乘的计算结果从CO1搬运到workspace Fixpipe(xGm, c1Local, fixpipeParams); outQueueCO1.FreeTensor(c1Local); } __aicore__ inline void CopyIn1() { PipeBarrier<PIPE_ALL>(); // 将矩阵乘的计算结果从workspace搬运到UB LocalTensor<float> src0Local = inQueueSrc0.AllocTensor<float>(); DataCopy(src0Local, xGm, cSize); inQueueSrc0.EnQue(src0Local); } __aicore__ inline void Compute1() { LocalTensor<float> src0Local = inQueueSrc0.DeQue<float>(); LocalTensor<half> tmpLocal = inQueueTmp.AllocTensor<half>(); LocalTensor<half> deqLocal = inQueueDeq.DeQue<half>(); LocalTensor<int8_t> dstLocal = outQueueDst.AllocTensor<int8_t>(); // 量化计算 Cast(tmpLocal, src0Local, RoundMode::CAST_NONE, cSize); LocalTensor<half> tmpHalfBuffer = src0Local.ReinterpretCast<half>(); Mul(tmpHalfBuffer, tmpLocal, deqLocal, cSize); Cast(dstLocal, tmpHalfBuffer, RoundMode::CAST_NONE, cSize); outQueueDst.EnQue<int8_t>(dstLocal); inQueueSrc0.FreeTensor(src0Local); inQueueTmp.FreeTensor(tmpLocal); inQueueDeq.FreeTensor(deqLocal); } __aicore__ inline void CopyOut1() { ... } private: TPipe pipe; TQue<QuePosition::A1, 1> inQueueA1; TQue<QuePosition::A2, 1> inQueueA2; TQue<QuePosition::B1, 1> inQueueB1; TQue<QuePosition::B2, 1> inQueueB2; TQue<QuePosition::CO1, 1> outQueueCO1; TQue<QuePosition::VECIN, 1> inQueueDeq; TQue<QuePosition::VECIN, 1> inQueueSrc0; TQue<QuePosition::VECCALC, 1> inQueueTmp; TQue<QuePosition::VECOUT, 1> outQueueDst; GlobalTensor<half> aGM; GlobalTensor<half> bGM; GlobalTensor<dst_T> cGM; GlobalTensor<float> biasGM; uint16_t m = 32, k = 32, n = 32; uint16_t aSize, bSize, cSize; ...
【正例】
该算子对矩阵乘的结果进行量化计算时,可将量化参数搬运到FB(Fixpipe Buffer)上,调用一次Fixpipe接口实现矩阵乘结果的量化计算。
... public: __aicore__ inline KernelSample() { aSize = m * k; bSize = k * n; cSize = m * n; } __aicore__ inline void Init(__gm__ uint8_t *a, __gm__ uint8_t *b, __gm__ uint8_t *c, __gm__ uint8_t *deqTensor) { aGM.SetGlobalBuffer((__gm__ half *)a); bGM.SetGlobalBuffer((__gm__ half *)b); cGM.SetGlobalBuffer((__gm__ float *)c); deqGM.SetGlobalBuffer((__gm__ uint64_t *)deqTensor); pipe.InitBuffer(inQueueA1, 1, aSize * sizeof(half)); pipe.InitBuffer(inQueueA2, 1, aSize * sizeof(half)); pipe.InitBuffer(inQueueB1, 1, bSize * sizeof(half)); pipe.InitBuffer(inQueueB2, 2, bSize * sizeof(half)); pipe.InitBuffer(outQueueCO1, 1, cSize * sizeof(float)); pipe.InitBuffer(inQueueDeq1, 1, cSize * sizeof(uint64_t)); pipe.InitBuffer(inQueueDeq, 1, cSize * sizeof(uint64_t)); } __aicore__ inline void Process() { CopyIn(); SplitA(); SplitB(); SplitDeq(); Compute(); CopyOut(); } private: __aicore__ inline void CopyIn() { LocalTensor<half> a1Local = inQueueA1.AllocTensor<half>(); LocalTensor<half> b1Local = inQueueB1.AllocTensor<half>(); LocalTensor<uint64_t> deq1Local = inQueueDeq1.AllocTensor<uint64_t>(); Nd2NzParams dataCopyA1Params; dataCopyA1Params.ndNum = 1; dataCopyA1Params.nValue = m; dataCopyA1Params.dValue = k; dataCopyA1Params.srcNdMatrixStride = 0; dataCopyA1Params.srcDValue = k; dataCopyA1Params.dstNzC0Stride = m; dataCopyA1Params.dstNzNStride = 1; dataCopyA1Params.dstNzMatrixStride = 0; DataCopy(a1Local, aGM, dataCopyA1Params); Nd2NzParams dataCopyB1Params; dataCopyB1Params.ndNum = 1; dataCopyB1Params.nValue = k; dataCopyB1Params.dValue = n; dataCopyB1Params.srcNdMatrixStride = 0; dataCopyB1Params.srcDValue = n; dataCopyB1Params.dstNzC0Stride = k; dataCopyB1Params.dstNzNStride = 1; dataCopyB1Params.dstNzMatrixStride = 0; DataCopy(b1Local, bGM, dataCopyB1Params); // 将量化参数搬运到L1上 DataCopy(deq1Local, deqGM, cSize); inQueueA1.EnQue(a1Local); inQueueB1.EnQue(b1Local); inQueueDeq.EnQue(deq1Local); } __aicore__ inline void SplitA() { ... } __aicore__ inline void SplitB() { ... } __aicore__ inline void SplitDeq() { LocalTensor<uint64_t> deq1Local = inQueueDeq1.DeQue<uint64_t>(); LocalTensor<uint64_t> deqLocal = inQueueDeq.AllocTensor<uint64_t>(); // 将量化参数从L1->FB DataCopy(deqLocal, deq1Local, { 1, (uint16_t)(cSize * sizeof(uint64_t) / 128), 0, 0 }); inQueueDeq.EnQue<uint61_t>(deqLocal); inQueueDeq1.FreeTensor(deq1Local); } __aicore__ inline void Compute() { LocalTensor<half> a2Local = inQueueA2.DeQue<half>(); LocalTensor<half> b2Local = inQueueB2.DeQue<half>(); LocalTensor<float> c1Local = outQueueCO1.AllocTensor<float>(); MmadParams mmadParams; mmadParams.m = m; mmadParams.n = n; mmadParams.k = k; // 矩阵乘 Mmad(c1Local, a2Local, b2Local, mmadParams); // m*n outQueueCO1.EnQue<float>(c1Local); inQueueA2.FreeTensor(a2Local); inQueueB2.FreeTensor(b2Local); } __aicore__ inline void CopyOut() { LocalTensor<float> c1Local = outQueueCO1.DeQue<float>(); LocalTensor<uint64_t> deqLocal = inQueueDeq.DeQue<uint64_t>(); SetFixpipeNz2ndFlag(1, 0, 0); DataCopyCO12DstParams dataCopyParams; dataCopyParams.nSize = n; dataCopyParams.mSize = m; dataCopyParams.srcStride = m; dataCopyParams.dstStride = n; dataCopyParams.quantPre = QuantMode_t::VQF322B8_PRE; dataCopyParams.nz2ndEn = true; // 将矩阵乘进行量化后的计算结果搬出 DataCopy(cGM, c1Local, DataCopyCO12DstParams); outQueueCO1.FreeTensor(c1Local); } private: TPipe pipe; TQue<QuePosition::A1, 1> inQueueA1; TQue<QuePosition::A2, 1> inQueueA2; TQue<QuePosition::B1, 1> inQueueB1; TQue<QuePosition::B2, 1> inQueueB2; TQue<QuePosition::C1, 1> inQueueDeq1; TQue<QuePosition::C2PIPE2GM, 1> inQueueDeq; TQue<QuePosition::CO1, 1> outQueueCO1; GlobalTensor<half> aGM; GlobalTensor<half> bGM; GlobalTensor<dst_T> cGM; GlobalTensor<uint64_t> deqTensorGM; uint16_t m = 32, k = 32, n = 32; uint16_t aSize, bSize, cSize; ...
父主题: 内存优化