简介
本节介绍基于Ascend IR定义的算子信息,在使用算子之前,请先阅读该章节所列的相关说明:
介绍算子规格中所列的Format信息。 |
|
详细介绍算子规格中所列的TensorType类型。 |
|
当部分算子(如Add、Mul等)输入的Tensor数据类型不一致时,算子内部计算会自动提升数据类型。该部分给出数据类型提升的规则。 |
|
列出确定性计算特性涉及和支持的算子。 |
Format
- ND:表示支持任意格式,仅有Square、Tanh等这些单输入对自身处理的算子外,其它需要慎用。
- NC1HWC0:自研的5维数据格式。其中,C0与微架构强相关,该值等于cube单元的size,例如16;C1是将C维度按照C0切分:C1=C/C0, 若结果不整除,最后一份数据需要padding到C0。
- FRACTAL_Z:卷积的权重的格式。
TensorType
struct TensorType { explicit TensorType(DataType dt); TensorType(const std::initializer_list<DataType> &types); static TensorType ALL() { return TensorType{DT_BOOL, DT_COMPLEX128, DT_COMPLEX64, DT_DOUBLE, DT_FLOAT, DT_FLOAT16, DT_INT16, DT_INT32, DT_INT64, DT_INT8, DT_QINT16, DT_QINT32, DT_QINT8, DT_QUINT16, DT_QUINT8, DT_RESOURCE, DT_STRING, DT_UINT16, DT_UINT32, DT_UINT64, DT_UINT8}; } static TensorType QuantifiedType() { return TensorType{DT_QINT16, DT_QINT32, DT_QINT8, DT_QUINT16, DT_QUINT8}; } static TensorType OrdinaryType() { return TensorType{DT_BOOL, DT_COMPLEX128, DT_COMPLEX64, DT_DOUBLE, DT_FLOAT, DT_FLOAT16, DT_INT16, DT_INT32, DT_INT64, DT_INT8, DT_UINT16, DT_UINT32, DT_UINT64, DT_UINT8}; } static TensorType BasicType() { return TensorType{DT_COMPLEX128, DT_COMPLEX64, DT_DOUBLE, DT_FLOAT, DT_FLOAT16, DT_INT16, DT_INT32, DT_INT64, DT_INT8, DT_QINT16, DT_QINT32, DT_QINT8, DT_QUINT16, DT_QUINT8, DT_UINT16, DT_UINT32, DT_UINT64, DT_UINT8}; } static TensorType NumberType() { return TensorType{DT_COMPLEX128, DT_COMPLEX64, DT_DOUBLE, DT_FLOAT, DT_FLOAT16, DT_INT16, DT_INT32, DT_INT64, DT_INT8, DT_QINT32, DT_QINT8, DT_QUINT8, DT_UINT16, DT_UINT32, DT_UINT64, DT_UINT8}; } static TensorType RealNumberType() { return TensorType{DT_DOUBLE, DT_FLOAT, DT_FLOAT16, DT_INT16, DT_INT32, DT_INT64, DT_INT8, DT_UINT16, DT_UINT32, DT_UINT64, DT_UINT8}; } static TensorType ComplexDataType() { return TensorType{DT_COMPLEX128, DT_COMPLEX64}; } static TensorType IntegerDataType() { return TensorType{DT_INT16, DT_INT32, DT_INT64, DT_INT8, DT_UINT16, DT_UINT32, DT_UINT64, DT_UINT8}; } static TensorType SignedDataType() { return TensorType{DT_INT16, DT_INT32, DT_INT64, DT_INT8}; } static TensorType UnsignedDataType() { return TensorType{DT_UINT16, DT_UINT32, DT_UINT64, DT_UINT8}; } static TensorType FloatingDataType() { return TensorType{DT_DOUBLE, DT_FLOAT, DT_FLOAT16}; } static TensorType IndexNumberType() { return TensorType{DT_INT32, DT_INT64}; } static TensorType UnaryDataType() { return TensorType{DT_COMPLEX128, DT_COMPLEX64, DT_DOUBLE, DT_FLOAT, DT_FLOAT16}; } static TensorType FLOAT() { return TensorType{DT_FLOAT, DT_FLOAT16}; } std::shared_ptr<TensorTypeImpl> tensor_type_impl_; };
Type Promotion
当部分算子(如Add、Mul等)输入的Tensor数据类型不一致时,算子内部计算会自动提升数据类型。数据类型提升的规则如下表:
数据类型 |
f32 |
f16 |
bf16 |
s8 |
u8 |
s16 |
u16 |
s32 |
u32 |
s64 |
u64 |
bool |
c32 |
c64 |
f32 |
f32 |
f32 |
f32 |
f32 |
f32 |
f32 |
× |
f32 |
× |
f32 |
× |
f32 |
c64 |
c64 |
f16 |
f32 |
f16 |
f32 |
f16 |
f16 |
f16 |
× |
f16 |
× |
f16 |
× |
f16 |
c32 |
c64 |
bf16 |
f32 |
f64 |
bf16 |
bf16 |
bf16 |
bf16 |
× |
bf16 |
× |
bf16 |
× |
bf16 |
c32 |
c64 |
s8 |
f32 |
f16 |
bf16 |
s8 |
s16 |
s16 |
× |
s32 |
× |
s64 |
× |
s8 |
c32 |
c64 |
u8 |
f32 |
f16 |
bf16 |
s16 |
u8 |
s16 |
× |
s32 |
× |
s64 |
× |
u8 |
c32 |
c64 |
s16 |
f32 |
f16 |
bf16 |
s16 |
s16 |
s16 |
× |
s32 |
× |
s64 |
× |
s16 |
c32 |
c64 |
u16 |
× |
× |
× |
× |
× |
× |
u16 |
× |
× |
× |
× |
× |
× |
× |
s32 |
f32 |
f16 |
bf16 |
s32 |
s32 |
s32 |
× |
s32 |
× |
s64 |
× |
s32 |
c32 |
c64 |
u32 |
× |
× |
× |
× |
× |
× |
× |
× |
u32 |
× |
× |
× |
× |
× |
s64 |
f32 |
f16 |
bf16 |
s64 |
s64 |
s64 |
× |
s64 |
× |
s64 |
× |
s64 |
c32 |
c64 |
u64 |
× |
× |
× |
× |
× |
× |
× |
× |
× |
× |
u64 |
× |
× |
× |
bool |
f32 |
f16 |
bf16 |
s8 |
u8 |
s16 |
× |
s32 |
× |
s64 |
× |
bool |
c32 |
c64 |
c32 |
c64 |
c32 |
c32 |
c32 |
c32 |
c32 |
× |
c32 |
× |
c32 |
× |
c32 |
c32 |
c64 |
c64 |
c64 |
c64 |
c64 |
c64 |
c64 |
c64 |
× |
c64 |
× |
c64 |
× |
c64 |
c64 |
c64 |
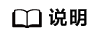
- 为方便描述,表格中使用的数据类型是简写形式,代表的含义:DT_FLOAT(f32)、DT_FLOAT16(f16)、DT_BF16(bf16)、DT_INT8(s8)、DT_UINT8(u8)、DT_INT16(s16)、DT_UINT16(u16)、DT_INT32(s32)、DT_UINT32(u32)、DT_INT64(s64)、DT_UINT64(u64)、DT_BOOL(bool)、DT_COMPLEX64(c32)、DT_COMPLEX64(c64)。
- 当前AI Core引擎的算子精度提升暂不支持DT_DOUBLE类型与DT_COMPLEX128类型。示例:当Mul算子输入参数的数据类型分别为(float32, double),无法提升成双double的输入走AI Core引擎,会分配到AI CPU引擎。
- 表格中表头和最左侧一列分别表示待推导的两个输入数据类型,表格对应位置表示推导出的数据类型。
- ×表示两种数据类型不能进行推导计算。
确定性计算
算子实现中,由于存在异步的多线程执行,会导致浮点数累加的顺序变化,算子在相同的硬件和输入下,多次执行的结果可能不同;当开启确定性计算功能时,算子在相同的硬件和输入下,多次执行将产生相同的输出。
如下针对确定性计算特性的算子,如果所列算子不在对应的规格清单中,则说明当前芯片版本不支持该算子。
- 如下算子涉及但未支持确定性计算:
- resizegradD
- WeightQuantBatchMatmulV2
- 如下算子涉及且已支持确定性计算:
- AvgPool3DGrad
- BatchMatMul
- BatchMatMulV2
- BiasAddGrad
- BinaryCrossEntropy
- BN3DTrainingReduce
- BN3DTrainingUpdateGrad
- BNTrainingReduce
- BNTrainingUpdateGrad
- Conv2DBackpropFilter
- Conv3DBackpropFilter
- EmbeddingDenseGrad
- FusedMulAddNL2loss
- FullyConnection
- GroupNormGrad
- Histogram
- IndexPut
- IndexPutV2
- InplaceIndexAdd
- KLDiv
- LayerNormBetaGammaBackpropV2
- LayerNormGradV3
- LpNormReduceV2
- LpNormV2
- MseLoss
- MatMul
- MatMulV2
- MseLoss
- NLLLoss
- ReduceMean
- ReduceMeanD
- ReduceSum
- ReduceSumD
- ScatterAdd
- ScatterElements
- ScatterNd
- ScatterNdAdd
- SquareSumV1
- UnsortedSegmentSum