Fixpipe
功能说明
矩阵计算完成后,对结果进行处理,例如对计算结果进行量化操作,并把数据从CO1搬迁到Global Memory中。
函数原型
- 传入FixpipeParamsV220
- 通路CO1->GM,不使能tensor量化功能:
1 2
template <typename DstT, typename SrcT, const FixpipeConfig& config = CFG_ROW_MAJOR> void Fixpipe(const GlobalTensor<DstT>& dstGlobal, const LocalTensor<SrcT>& srcLocal, const FixpipeParamsV220& intriParams)
- 通路CO1->GM,使能tensor量化功能:
1 2
template <typename DstT, typename SrcT, const FixpipeConfig& config = CFG_ROW_MAJOR, typename BufT = uint64_t, typename std::enable_if<IsSameType<PrimT<BufT>, uint64_t>::value, bool>::type = true> __aicore__ inline void Fixpipe(const GlobalTensor<DstT>& dstGlobal, const LocalTensor<SrcT>& srcLocal, const LocalTensor<BufT>& cbufWorkspace, const FixpipeParamsV220& intriParams);
- 通路CO1->GM,不使能tensor量化功能:
- 传入FixpipeParamsM300
- 通路CO1->UB,不使能tensor量化功能:
1 2
template <typename DstT, typename SrcT, const FixpipeConfig& config = CFG_ROW_MAJOR> void Fixpipe(const LocalTensor<DstT>& dstGlobal, const LocalTensor<SrcT>& srcLocal, const FixpipeParamsM300& intriParams)
- 通路CO1->UB,使能tensor量化功能:
1 2
template <typename DstT, typename SrcT, const FixpipeConfig& config = CFG_ROW_MAJOR, typename BufT = uint64_t, typename std::enable_if<IsSameType<PrimT<BufT>, uint64_t>::value, bool>::type = true> __aicore__ inline void Fixpipe(const LocalTensor<DstT>& dstLocal, const LocalTensor<SrcT>& srcLocal, const LocalTensor<BufT>& cbufWorkspace, const FixpipeParamsM300& intriParams);
- 通路CO1->GM,不使能tensor量化功能:
1 2
template <typename DstT, typename SrcT, const FixpipeConfig& config = CFG_ROW_MAJOR> void Fixpipe(const GlobalTensor<DstT>& dstGlobal, const LocalTensor<SrcT>& srcLocal, const FixpipeParamsM300& intriParams)
- 通路CO1->GM,使能tensor量化功能:
1 2
template <typename DstT, typename SrcT, const FixpipeConfig& config = CFG_ROW_MAJOR, typename BufT = uint64_t, typename std::enable_if<IsSameType<PrimT<BufT>, uint64_t>::value, bool>::type = true> __aicore__ inline void Fixpipe(const GlobalTensor<DstT>& dstGlobal, const LocalTensor<SrcT>& srcLocal, const LocalTensor<BufT>& cbufWorkspace, const FixpipeParamsM300& intriParams)
- 通路CO1->UB,不使能tensor量化功能:
参数说明
参数名 |
描述 |
||
---|---|---|---|
DstT |
目的操作数数据类型。 |
||
SrcT |
源操作数数据类型。 |
||
config |
Fixpipe相关配置参数,类型为FixpipeConfig。取值如下:
|
||
BufT |
cbufWorkspace的数据类型。
BufT后一个模板参数仅用于上述数据类型检查,用户无需关注。 |
参数名称 |
输入/输出 |
含义 |
||
---|---|---|---|---|
dstGlobal |
输出 |
目的操作数,类型为GlobalTensor。支持的数据类型为half/bfloat16_t/float/int32_t/int8_t/uint8_t。 数据格式为NZ或ND格式。经过fixpipe处理,在量化操作之后,会将矩阵计算中多申请的数据删除。 |
||
srcLocal |
输入 |
源操作数,支持的QuePosition为CO1,为Mmad接口计算的结果,类型为LocalTensor数据结构的定义请参考LocalTensor。支持的数据类型为float/int32_t,支持的QuePosition为CO1,数据格式为NZ格式。起始地址需要满足64B对齐。 |
||
intriParams |
输入 |
Fixpipe搬运参数,具体请参考表2 FixpipeParamsV220 结构体内参数定义。FixpipeParamsV220/FixpipeParamsM300结构体定义一致,定义如下:
|
||
cbufWorkspace |
输入 |
量化参数,类型为LocalTensor<uint64_t>,支持的QuePosition为A1。仅当quantPre为VDEQF16/VQF322B8_PRE/VREQ8时支持,quantPre介绍请参考FixpipeParamsV220/FixpipeParamsM300结构体中quantPre部分。 |
参数名称 |
数据类型 |
含义 |
||
---|---|---|---|---|
nSize |
输入 |
源NZ矩阵在N方向上的大小。 |
||
mSize |
输入 |
源NZ矩阵在M方向上的大小。 |
||
srcStride |
输入 |
源NZ矩阵中相邻Z排布的起始地址偏移,取值范围:srcStride∈[0, 65535], 单位:C0_Size(16*sizeof(T),T为srcLocal的数据类型)。 |
||
dstStride |
输入 |
|||
quantPre |
输入 |
默认值为QuantMode_t::NoQuant,即不使能量化功能。 QuantMode_t是一个枚举类型,用以控制量化模式,具体定义为:
|
||
deqScalar |
输入 |
scalar量化参数,表示单个scale值,支持的数据类型为uint64_t,仅当mode为DEQF16/QF322B8_PRE/REQ8时支持。 注:当mode为F322F16/F322BF16时不需要设置deqScalar。 |
||
ndNum |
输入 |
源NZ矩阵的数目,也就是传输ND矩阵的数目,取值范围:ndNum∈[1, 65535] |
||
srcNdStride |
输入 |
不同NZ矩阵起始地址之间的间隔,取值范围:srcNdStride∈[1, 512],单位:1024B。当ndNum配置为1时,srcNdStride配置为0即可,不生效。 |
||
dstNdStride |
输入 |
目的相邻ND矩阵起始地址之间的偏移,取值范围:dstNdstride∈[1, 65535],单位:element。当ndNum配置为1时,dstNdStride配置为0即可,不生效。 |
||
reluEn |
输入 |
是否使能relu的开关,false:不使能relu功能;true:使能relu功能。 |
||
unitFlag |
输入 |
预留参数,用户无需关心,使用默认值0即可。 |
||
isChannelSplit |
输入 |
是否使能通道拆分的功能。默认为false,不使能该功能。仅在src和dst都为float时才能使能通道拆分,且不能同时使能ChannelSplit和NZ2ND功能。 |
不使能NZ2ND的情况下,参数设置示例(通过Fixpipe接口搬运并去除dummy数据)和解释说明如下:
- nSize = 48,表示源NZ矩阵中待搬运矩阵(图中蓝色区域)在N方向上的大小为48个元素。
- mSize = 24,表示源NZ矩阵中待搬运矩阵在M方向上的大小为24个元素。
- srcStride = 64,表示源NZ矩阵中待搬运矩阵相邻Z排布的起始地址偏移,即下图中第一个蓝色Z排布的起始地址与第二个蓝色Z排布的起始地址之间的间隔为64 * C0_Size。
- dstStride = 40,表示目的NZ矩阵中相邻Z排布的起始地址偏移,即下图中第一个蓝色Z排布的起始地址与第二个蓝色Z排布的起始地址之间的间隔为40 * 32B。
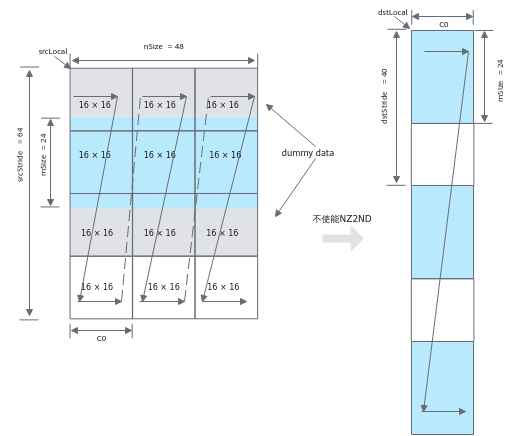
使能NZ2ND的情况下,参数设置示例和解释说明如下:
- ndNum = 2,表示源NZ矩阵的数目为2。图中蓝色区域为NZ矩阵1,紫色区域为NZ矩阵2。
- nSize = 32,表示源NZ矩阵(图中蓝色区域)在N方向上的大小为32个元素。
- mSize = 48,表示源NZ矩阵在M方向上的大小为48个元素。
- srcStride = 64,表示源NZ矩阵中相邻Z排布的起始地址偏移,即下图中第一个蓝色Z排布的起始地址与第二个蓝色Z排布的起始地址之间的间隔为64 * C0_Size。
- dstStride = 64,表示目的ND矩阵每一行中的元素个数为64。
- srcNdStride = 16, 表示不同NZ矩阵起始地址之间的间隔为16 *1024B。
- dstNdStride = 4096,表示目的相邻ND矩阵起始地址之间的偏移为4096个元素。
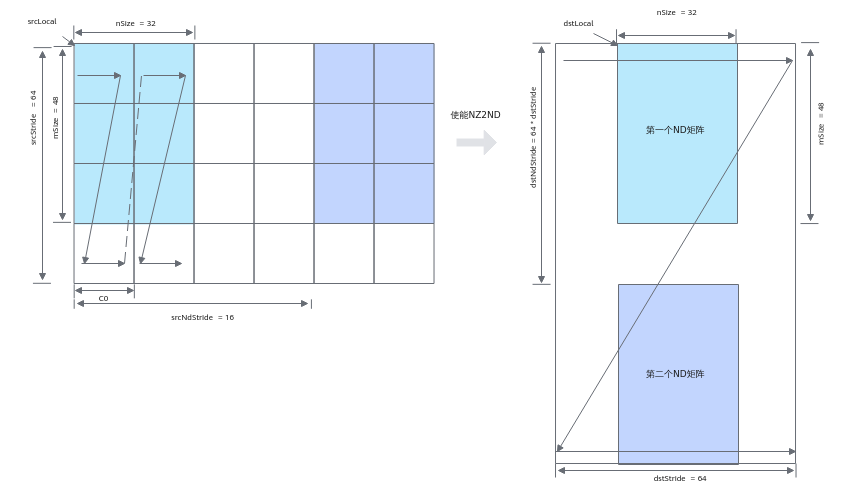
支持的型号
注意事项
- ndNum=0 表示不执行,此指令将视为NOP并报warning。
- 对于量化输入为float32数据类型的说明如下:
- 标准的IEEE 754 float32格式为:1bit符号位,8bits指数位,23bits尾数位;当前AI处理器支持的float32格式为:1bit符号位,8bits指数位,10bits尾数位。
- 如果用户提供的是标准的IEEE 754 float32输入,API内部会处理成处理器支持的float32格式进行计算,此时如果golden数据生成过程中使用的是标准的IEEE 754 float32数据,则可能引入精度不匹配问题,需要修正golden数据的生成,将量化参数的23bits尾数位的低13bits数据位清零再参与量化计算。
调用示例
- 示例一:通路CO1->GM,不使能tensor量化功能接口。输入A矩阵和B矩阵的数据类型为half,输出C矩阵为half,默认配置使能Nz2Nd的格式转换,使能F322F16量化将mmad计算出的结果由float量化成half。
1 2 3 4 5 6 7 8 9 10 11 12 13 14 15 16 17 18 19 20 21 22 23 24 25 26 27 28 29 30 31 32 33 34 35 36 37 38 39 40 41 42 43 44 45 46 47 48 49 50 51 52 53 54 55 56 57 58 59 60 61 62 63 64 65 66 67 68 69 70 71 72 73 74 75 76 77 78 79 80 81 82 83 84 85 86 87 88 89 90 91 92 93 94 95 96 97 98 99 100 101 102 103 104 105 106 107 108 109 110 111 112 113 114 115 116 117 118 119 120 121 122 123 124 125 126 127 128 129 130 131 132 133 134 135 136 137 138 139 140 141 142 143 144 145 146 147 148 149 150 151 152 153 154 155 156 157 158 159 160 161 162 163 164 165
#ifdef ASCENDC_CPU_DEBUG #include "tikicpulib.h" #endif #include "kernel_operator.h" template <typename C_T, typename A_T, typename B_T, typename dstCO1_T> class KernelMatmul { public: __aicore__ inline KernelMatmul(uint16_t mIn, uint8_t kIn, uint8_t nIn) { m = mIn; k = kIn; n = nIn; aSize = m * k; bSize = k * n; cSize = m * n; mBlocks = m / AscendC::BLOCK_CUBE; nBlocks = n / AscendC::BLOCK_CUBE; kBlocks = k / (AscendC::ONE_BLK_SIZE / sizeof(A_T)); } __aicore__ inline void Init(__gm__ uint8_t *a, __gm__ uint8_t *b, __gm__ uint8_t *c) { aGM.SetGlobalBuffer((__gm__ A_T *)a); bGM.SetGlobalBuffer((__gm__ B_T *)b); cGM.SetGlobalBuffer((__gm__ C_T *)c); pipe.InitBuffer(inQueueA1, 1, aSize * sizeof(A_T)); pipe.InitBuffer(inQueueA2, 1, aSize * sizeof(A_T)); pipe.InitBuffer(inQueueB1, 1, bSize * sizeof(B_T)); pipe.InitBuffer(inQueueB2, 2, bSize * sizeof(B_T)); pipe.InitBuffer(outQueueCO1, 1, cSize * sizeof(dstCO1_T)); } __aicore__ inline void Process() { CopyIn(); SplitA(); SplitB(); Compute(); CopyOut(); } private: __aicore__ inline void CopyIn() { AscendC::LocalTensor<A_T> a1Local = inQueueA1.AllocTensor<A_T>(); AscendC::LocalTensor<B_T> b1Local = inQueueB1.AllocTensor<B_T>(); AscendC::Nd2NzParams dataCopyA1Params; dataCopyA1Params.ndNum = 1; dataCopyA1Params.nValue = m; dataCopyA1Params.dValue = k; dataCopyA1Params.srcNdMatrixStride = 0; dataCopyA1Params.srcDValue = k; dataCopyA1Params.dstNzC0Stride = m; dataCopyA1Params.dstNzNStride = 1; dataCopyA1Params.dstNzMatrixStride = 0; AscendC::Nd2NzParams dataCopyB1Params; dataCopyB1Params.ndNum = 1; dataCopyB1Params.nValue = k; dataCopyB1Params.dValue = n; dataCopyB1Params.srcNdMatrixStride = 0; dataCopyB1Params.srcDValue = n; dataCopyB1Params.dstNzC0Stride = k; dataCopyB1Params.dstNzNStride = 1; dataCopyB1Params.dstNzMatrixStride = 0; // AscendC::DataCopy GM->L1:ND->大N小z AscendC::DataCopy(a1Local, aGM, dataCopyA1Params); AscendC::DataCopy(b1Local, bGM, dataCopyB1Params); inQueueA1.EnQue(a1Local); inQueueB1.EnQue(b1Local); } __aicore__ inline void SplitA() { AscendC::LocalTensor<A_T> a1Local = inQueueA1.DeQue<A_T>(); AscendC::LocalTensor<A_T> a2Local = inQueueA2.AllocTensor<A_T>(); // AscendC::LoadData L1->L0A AscendC::LoadData2dParams loadL0AParams; loadL0AParams.repeatTimes = mBlocks; loadL0AParams.srcStride = 1; loadL0AParams.dstGap = kBlocks - 1; loadL0AParams.ifTranspose = false; for (int i = 0; i < kBlocks; i++) { AscendC::LoadData(a2Local[i * 16 * (32 / sizeof(A_T))], a1Local[i * m * (32 / sizeof(A_T))], loadL0AParams); } inQueueA2.EnQue<A_T>(a2Local); inQueueA1.FreeTensor(a1Local); } __aicore__ inline void SplitB() { AscendC::LocalTensor<B_T> b1Local = inQueueB1.DeQue<B_T>(); AscendC::LocalTensor<B_T> b2Local = inQueueB2.AllocTensor<B_T>(); // Load2d transpose L1->L0B AscendC::LoadData2dTransposeParams loadDataParams; loadDataParams.startIndex = 0; loadDataParams.srcStride = 1; loadDataParams.addrMode = 0; loadDataParams.repeatTimes = k * n / B32_B16_SIZE; loadDataParams.dstGap = 0; loadDataParams.dstFracGap = n / n_block - 1; AscendC::LoadDataWithTranspose(b2Local, b1Local, loadDataParams); inQueueB1.FreeTensor(b1Local); inQueueB2.EnQue<B_T>(b2Local); } __aicore__ inline void Compute() { AscendC::LocalTensor<A_T> a2Local = inQueueA2.DeQue<A_T>(); AscendC::LocalTensor<B_T> b2Local = inQueueB2.DeQue<B_T>(); AscendC::LocalTensor<dstCO1_T> c1Local = outQueueCO1.AllocTensor<dstCO1_T>(); AscendC::MmadParams mmadParams; mmadParams.m = m; mmadParams.n = n; mmadParams.k = k; AscendC::Mmad(c1Local, a2Local, b2Local, mmadParams); // m*n outQueueCO1.EnQue<dstCO1_T>(c1Local); inQueueA2.FreeTensor(a2Local); inQueueB2.FreeTensor(b2Local); } __aicore__ inline void CopyOut() { AscendC::LocalTensor<dstCO1_T> c1Local = outQueueCO1.DeQue<dstCO1_T>(); AscendC::FixpipeParamsV220 fixpipeParams; fixpipeParams.nSize = n; fixpipeParams.mSize = m; fixpipeParams.srcStride = m; fixpipeParams.dstStride = n; fixpipeParams.ndNum = 1; fixpipeParams.srcNdStride = 2; fixpipeParams.dstNdStride = m*n; fixpipeParams.quantPre = QuantMode_t::F322F16; AscendC::Fixpipe(cGM, c1Local, fixpipeParams); outQueueCO1.FreeTensor(c1Local); } private: AscendC::TPipe pipe; AscendC::TQue<AscendC::QuePosition::A1, 1> inQueueA1; AscendC::TQue<AscendC::QuePosition::A2, 1> inQueueA2; AscendC::TQue<AscendC::QuePosition::B1, 1> inQueueB1; AscendC::TQue<AscendC::QuePosition::B2, 1> inQueueB2; AscendC::TQue<AscendC::QuePosition::CO1, 1> outQueueCO1; AscendC::GlobalTensor<A_T> aGM; AscendC::GlobalTensor<B_T> bGM; AscendC::GlobalTensor<C_T> cGM; uint16_t m, k, n; uint16_t B32_B16_SIZE = 16 * 16; uint8_t n_block = 16; uint16_t aSize, bSize, cSize, mBlocks, nBlocks, kBlocks; }; #define KERNEL_MATMUL(c_type, a_type, b_type, co1_type, mIn, kIn, nIn) \ extern "C" __global__ __aicore__ void cube_matmul_loaddata_operator( \ __gm__ uint8_t *a, __gm__ uint8_t *b, __gm__ uint8_t *c) \ { \ if (g_coreType == AscendC::AIV) { \ return; \ } \ KernelMatmul<c_type, a_type, b_type, co1_type> op(mIn, kIn, nIn);\ op.Init(a, b, c); \ op.Process(); \ } KERNEL_MATMUL(half, half, half, float, 32, 32, 16);
示例结果:输入数据A矩阵: [[6. 3. 9. 4. 5. 3. 9. 7. 3. 6. 2. 7. 3. 8. 8. 1. 8. 8. 5. 6. 6. 8. 2. 2. 3. 6. 4. 8. 9. 6. 6. 1.] [2. 5. 7. 2. 4. 2. 5. 2. 4. 6. 4. 8. 5. 7. 1. 4. 3. 1. 8. 6. 4. 6. 9. 1. 8. 2. 9. 5. 3. 7. 7. 8.] [5. 8. 2. 1. 4. 5. 7. 7. 4. 6. 8. 5. 6. 5. 4. 2. 5. 4. 7. 9. 5. 4. 7. 4. 2. 2. 1. 7. 8. 4. 6. 6.] [8. 2. 4. 7. 6. 9. 7. 7. 4. 5. 6. 7. 6. 6. 5. 3. 7. 6. 7. 4. 5. 4. 1. 9. 6. 7. 8. 9. 4. 9. 5. 5.] [4. 9. 4. 2. 7. 8. 3. 4. 1. 5. 3. 8. 8. 5. 5. 8. 3. 8. 5. 3. 9. 4. 5. 4. 2. 4. 3. 8. 9. 8. 4. 3.] [1. 3. 8. 3. 1. 9. 9. 5. 5. 6. 3. 2. 3. 4. 3. 3. 5. 9. 6. 7. 1. 3. 4. 2. 8. 5. 9. 1. 9. 5. 8. 9.] [3. 3. 1. 3. 5. 2. 7. 8. 8. 9. 6. 9. 3. 6. 5. 5. 2. 3. 2. 3. 5. 1. 6. 1. 7. 8. 7. 2. 2. 7. 8. 1.] [4. 4. 6. 4. 6. 5. 1. 2. 7. 8. 3. 2. 9. 9. 7. 7. 7. 1. 2. 7. 2. 1. 5. 2. 1. 3. 2. 1. 3. 3. 2. 9.] [4. 6. 3. 5. 8. 4. 1. 1. 2. 5. 8. 8. 8. 3. 9. 6. 5. 6. 7. 9. 2. 1. 9. 3. 2. 5. 4. 1. 7. 5. 3. 9.] [7. 2. 3. 4. 9. 5. 6. 3. 4. 5. 4. 7. 4. 1. 9. 4. 2. 1. 7. 4. 9. 2. 4. 5. 4. 5. 8. 7. 2. 2. 8. 3.] [5. 7. 6. 2. 9. 4. 7. 1. 8. 6. 2. 1. 6. 5. 5. 6. 3. 8. 1. 5. 2. 1. 8. 3. 1. 9. 3. 3. 5. 2. 2. 5.] [4. 7. 5. 9. 9. 6. 7. 3. 1. 9. 2. 6. 5. 2. 6. 7. 1. 7. 6. 9. 3. 7. 6. 1. 3. 9. 2. 4. 1. 9. 4. 8.] [2. 4. 3. 1. 1. 2. 2. 7. 2. 3. 7. 9. 8. 8. 3. 4. 1. 2. 9. 2. 9. 4. 4. 8. 5. 7. 7. 3. 9. 9. 5. 3.] [3. 1. 1. 6. 1. 8. 3. 3. 6. 3. 4. 4. 3. 8. 2. 1. 1. 1. 6. 5. 8. 8. 5. 8. 5. 1. 2. 2. 1. 3. 7. 4.] [4. 2. 8. 4. 4. 1. 9. 6. 9. 9. 5. 4. 3. 1. 3. 8. 1. 2. 8. 2. 5. 8. 9. 3. 2. 5. 9. 7. 7. 4. 2. 1.] [2. 6. 7. 1. 3. 9. 9. 9. 6. 4. 5. 8. 1. 3. 7. 3. 8. 7. 3. 4. 8. 6. 9. 6. 8. 9. 4. 4. 7. 6. 1. 4.] [2. 8. 2. 1. 2. 6. 2. 8. 5. 9. 9. 8. 6. 4. 4. 1. 4. 1. 4. 4. 4. 7. 5. 9. 9. 8. 9. 1. 8. 4. 7. 3.] [3. 6. 2. 5. 1. 2. 9. 2. 6. 7. 4. 5. 9. 6. 5. 9. 7. 9. 5. 5. 6. 7. 4. 7. 7. 6. 3. 6. 5. 2. 8. 3.] [1. 7. 3. 2. 4. 8. 1. 7. 3. 4. 1. 6. 1. 4. 4. 1. 6. 7. 9. 3. 9. 2. 2. 2. 2. 8. 1. 1. 6. 3. 6. 1.] [4. 3. 9. 5. 2. 2. 1. 8. 5. 8. 9. 2. 4. 3. 2. 1. 8. 6. 6. 2. 9. 2. 9. 3. 9. 5. 3. 7. 9. 7. 6. 2.] [9. 4. 8. 1. 3. 7. 9. 5. 2. 4. 9. 9. 6. 9. 6. 4. 6. 3. 3. 9. 6. 8. 1. 5. 5. 1. 6. 5. 1. 9. 3. 9.] [2. 5. 2. 1. 8. 9. 9. 8. 1. 6. 1. 1. 9. 8. 3. 5. 6. 4. 2. 1. 3. 7. 8. 9. 6. 6. 1. 9. 1. 7. 6. 8.] [4. 7. 6. 6. 2. 2. 1. 8. 7. 1. 1. 2. 1. 1. 9. 8. 9. 4. 9. 5. 7. 8. 9. 9. 5. 1. 6. 8. 9. 6. 7. 5.] [1. 1. 6. 9. 9. 3. 7. 6. 5. 6. 5. 1. 5. 5. 3. 7. 6. 7. 4. 8. 8. 2. 2. 5. 7. 8. 8. 2. 9. 1. 5. 1.] [5. 4. 6. 8. 8. 3. 7. 7. 5. 7. 8. 7. 4. 8. 2. 9. 4. 8. 1. 3. 8. 5. 3. 7. 3. 7. 1. 9. 1. 5. 4. 7.] [6. 3. 1. 2. 8. 3. 2. 6. 8. 2. 8. 4. 1. 9. 4. 7. 5. 1. 7. 5. 5. 1. 1. 1. 2. 8. 1. 7. 9. 8. 5. 4.] [2. 8. 5. 1. 3. 4. 9. 8. 6. 9. 6. 2. 4. 2. 2. 7. 8. 2. 1. 3. 7. 1. 4. 6. 4. 6. 3. 3. 1. 6. 8. 3.] [5. 1. 5. 5. 9. 7. 9. 2. 1. 4. 7. 8. 1. 9. 8. 1. 2. 4. 3. 9. 9. 6. 7. 9. 1. 5. 1. 9. 2. 5. 6. 9.] [1. 9. 9. 6. 5. 7. 9. 5. 4. 1. 2. 8. 3. 8. 1. 9. 6. 1. 7. 9. 3. 2. 2. 4. 7. 9. 9. 4. 7. 1. 5. 8.] [3. 2. 2. 5. 9. 3. 6. 9. 2. 4. 4. 8. 4. 2. 6. 1. 2. 8. 8. 8. 9. 7. 7. 1. 9. 6. 5. 8. 3. 3. 3. 4.] [9. 1. 6. 1. 3. 7. 8. 1. 2. 6. 5. 9. 4. 4. 7. 2. 3. 9. 8. 7. 8. 2. 6. 4. 5. 6. 5. 4. 9. 6. 1. 9.] [4. 3. 2. 7. 8. 1. 7. 2. 9. 7. 7. 4. 2. 8. 2. 5. 6. 9. 5. 1. 3. 9. 8. 2. 4. 8. 4. 7. 4. 1. 3. 7.]] 输入数据B矩阵: [[3. 5. 9. 6. 2. 9. 3. 6. 5. 9. 5. 5. 3. 8. 5. 2.] [5. 1. 5. 7. 5. 4. 2. 2. 4. 8. 1. 1. 3. 3. 7. 2.] [6. 7. 4. 6. 1. 4. 8. 3. 9. 2. 2. 3. 4. 6. 5. 3.] [4. 8. 2. 6. 4. 8. 6. 7. 3. 8. 6. 7. 3. 8. 1. 1.] [6. 7. 8. 6. 1. 9. 9. 3. 9. 9. 2. 1. 3. 3. 3. 3.] [7. 2. 4. 7. 5. 8. 9. 2. 1. 7. 9. 6. 8. 7. 1. 3.] [3. 3. 9. 2. 3. 9. 4. 1. 8. 2. 5. 1. 2. 6. 5. 5.] [6. 4. 8. 8. 7. 5. 9. 6. 7. 6. 8. 8. 2. 6. 1. 2.] [4. 2. 3. 8. 6. 1. 1. 1. 7. 9. 5. 2. 2. 5. 7. 6.] [4. 5. 9. 5. 6. 8. 1. 2. 1. 9. 2. 7. 8. 6. 6. 1.] [4. 8. 6. 6. 3. 1. 7. 8. 7. 3. 2. 9. 8. 6. 9. 8.] [3. 2. 5. 5. 7. 9. 7. 7. 4. 8. 3. 5. 2. 7. 1. 2.] [3. 8. 2. 8. 9. 5. 1. 5. 7. 4. 1. 3. 4. 1. 4. 6.] [9. 5. 2. 2. 4. 6. 3. 3. 7. 1. 9. 6. 8. 6. 4. 7.] [2. 3. 8. 1. 5. 9. 8. 4. 5. 4. 6. 5. 4. 5. 3. 2.] [3. 5. 4. 2. 1. 2. 9. 2. 3. 8. 9. 8. 8. 1. 2. 7.] [1. 4. 5. 1. 3. 8. 2. 5. 9. 9. 5. 5. 5. 6. 4. 2.] [7. 6. 7. 7. 6. 9. 1. 3. 8. 1. 9. 8. 8. 5. 1. 6.] [5. 3. 8. 9. 8. 2. 6. 6. 1. 3. 2. 1. 2. 9. 3. 9.] [1. 1. 4. 9. 8. 6. 6. 5. 6. 8. 4. 2. 2. 7. 2. 1.] [8. 1. 3. 5. 8. 7. 5. 7. 4. 6. 7. 4. 8. 2. 2. 3.] [5. 8. 6. 8. 1. 8. 6. 8. 3. 9. 1. 1. 3. 8. 3. 2.] [7. 7. 5. 1. 5. 4. 6. 1. 1. 6. 8. 8. 1. 7. 7. 2.] [1. 7. 7. 7. 7. 6. 1. 7. 3. 3. 8. 9. 3. 8. 9. 8.] [4. 9. 5. 6. 9. 6. 8. 9. 1. 1. 6. 5. 1. 4. 3. 5.] [4. 1. 8. 9. 6. 5. 5. 7. 8. 9. 8. 2. 7. 5. 5. 3.] [9. 8. 4. 9. 5. 4. 7. 5. 7. 6. 9. 8. 5. 7. 2. 9.] [6. 6. 5. 1. 4. 5. 9. 6. 7. 5. 5. 2. 3. 7. 6. 5.] [5. 2. 5. 7. 9. 2. 2. 3. 2. 3. 1. 4. 6. 5. 3. 1.] [5. 1. 9. 3. 2. 4. 1. 6. 7. 7. 4. 9. 8. 8. 6. 1.] [3. 7. 5. 6. 7. 8. 2. 2. 8. 7. 6. 1. 3. 5. 3. 2.] [7. 6. 7. 8. 6. 5. 2. 2. 8. 2. 2. 6. 6. 4. 9. 6.]] 输出数据C矩阵: [[ 807. 767. 1007. 925. 853. 1079. 837. 782. 977. 960. 838. 746. 767. 1013. 642. 594.] [ 778. 775. 850. 874. 801. 853. 767. 682. 808. 852. 719. 709. 651. 891. 663. 635.] [ 734. 705. 927. 901. 865. 906. 742. 687. 840. 892. 725. 718. 692. 911. 702. 601.] [ 877. 895. 1099. 1070. 954. 1136. 926. 912. 1028. 1057. 983. 930. 859. 1119. 760. 768.] [ 818. 722. 931. 904. 857. 969. 809. 724. 846. 948. 812. 786. 811. 885. 644. 619.] [ 780. 750. 907. 964. 865. 905. 738. 638. 861. 808. 816. 759. 735. 913. 627. 640.] [ 697. 671. 865. 810. 780. 863. 729. 656. 803. 892. 798. 734. 664. 819. 593. 561.] [ 619. 633. 716. 734. 667. 767. 612. 515. 749. 794. 641. 652. 650. 705. 596. 518.] [ 716. 738. 908. 907. 838. 902. 767. 684. 829. 907. 726. 787. 728. 872. 671. 609.] [ 692. 710. 876. 838. 779. 926. 812. 692. 791. 894. 767. 660. 629. 844. 588. 597.] [ 671. 639. 812. 787. 684. 815. 637. 511. 806. 819. 714. 627. 652. 734. 628. 546.] [ 779. 764. 1011. 962. 806. 1042. 845. 728. 883. 1027. 794. 762. 764. 949. 667. 576.] [ 750. 690. 856. 907. 875. 801. 716. 772. 771. 803. 760. 772. 724. 865. 633. 656.] [ 598. 605. 649. 731. 678. 741. 591. 593. 577. 694. 662. 591. 536. 750. 508. 508.] [ 754. 750. 902. 869. 746. 815. 807. 669. 780. 912. 750. 719. 658. 905. 658. 633.] [ 844. 758. 1037. 971. 920. 1038. 903. 800. 920. 983. 937. 863. 791. 1011. 726. 648.] [ 754. 782. 935. 1018. 936. 909. 770. 795. 799. 947. 796. 811. 726. 937. 708. 644.] [ 744. 828. 940. 936. 914. 1014. 753. 760. 893. 946. 874. 777. 768. 920. 699. 706.] [ 615. 467. 719. 754. 714. 750. 601. 560. 637. 739. 650. 544. 598. 699. 434. 437.] [ 785. 791. 906. 889. 868. 866. 766. 768. 836. 871. 787. 814. 738. 920. 693. 592.] [ 814. 822. 1006. 963. 831. 1062. 868. 826. 991. 950. 834. 853. 809. 1021. 745. 700.] [ 782. 812. 957. 847. 800. 998. 773. 688. 882. 890. 854. 770. 730. 889. 721. 642.] [ 792. 815. 966. 947. 895. 942. 858. 786. 859. 995. 884. 827. 701. 1006. 711. 657.] [ 758. 791. 878. 960. 861. 938. 818. 735. 889. 906. 861. 763. 751. 869. 588. 649.] [ 830. 853. 990. 936. 817. 1044. 862. 796. 990. 994. 902. 865. 834. 953. 744. 698.] [ 679. 586. 833. 792. 716. 754. 713. 653. 816. 856. 708. 654. 698. 802. 608. 566.] [ 636. 642. 844. 775. 723. 821. 652. 600. 809. 864. 743. 693. 671. 763. 652. 546.] [ 804. 789. 987. 887. 824. 1084. 868. 766. 933. 924. 859. 786. 762. 1002. 735. 639.] [ 813. 765. 906. 1016. 889. 947. 902. 735. 933. 949. 870. 738. 737. 943. 664. 708.] [ 790. 769. 946. 935. 877. 996. 899. 798. 840. 903. 807. 718. 651. 919. 579. 605.] [ 803. 725. 1003. 949. 900. 1002. 792. 749. 860. 863. 818. 812. 790. 972. 686. 657.] [ 787. 813. 910. 873. 751. 927. 751. 688. 874. 914. 795. 733. 721. 903. 697. 664.]]
- 示例二:通路CO1->GM,使能tensor量化功能接口。输入A矩阵和B矩阵的数据类型为int8,输出C矩阵为half,默认配置使能Nz2Nd的格式转换,使能tensor量化(VDEQF16)将mmad计算出的结果由int32 量化成half。
1 2 3 4 5 6 7 8 9 10 11 12 13 14 15 16 17 18 19 20 21 22 23 24 25 26 27 28 29 30 31 32 33 34 35 36 37 38 39 40 41 42 43 44 45 46 47 48 49 50 51 52 53 54 55 56 57 58 59 60 61 62 63 64 65 66 67 68 69 70 71 72 73 74 75 76 77 78 79 80 81 82 83 84 85 86 87 88 89 90 91 92 93 94 95 96 97 98 99 100 101 102 103 104 105 106 107 108 109 110 111 112 113 114 115 116 117 118 119 120 121 122 123 124 125 126 127 128 129 130 131 132 133 134 135 136 137 138 139 140 141 142 143 144 145 146 147 148 149 150 151 152 153 154 155 156 157 158 159 160 161 162 163 164 165 166 167 168 169 170 171 172 173 174 175 176 177 178 179 180 181 182 183 184 185
#ifdef ASCENDC_CPU_DEBUG #include "tikicpulib.h" #endif #include "kernel_operator.h" template <typename c_T, typename a_T, typename b_T, typename dstCO1_T> class KernelMatmul { public: __aicore__ inline KernelMatmul(uint16_t mIn, uint8_t kIn, uint8_t nIn) { m = mIn; k = kIn; n = nIn; aSize = m * k; bSize = k * n; cSize = m * n; mBlocks = m / AscendC::BLOCK_CUBE; nBlocks = n / AscendC::BLOCK_CUBE; kBlocks = k / (AscendC::ONE_BLK_SIZE / sizeof(a_T)); deqTensorLen = n; } __aicore__ inline void Init(__gm__ uint8_t *a, __gm__ uint8_t *b, __gm__ uint8_t *c, __gm__ uint8_t *deqTensor) { aGM.SetGlobalBuffer((__gm__ a_T *)a); bGM.SetGlobalBuffer((__gm__ b_T *)b); cGM.SetGlobalBuffer((__gm__ c_T *)c); deqTensorGM.SetGlobalBuffer((__gm__ uint64_t *)deqTensor); pipe.InitBuffer(inQueueA1, 1, aSize * sizeof(a_T)); pipe.InitBuffer(inQueueA2, 1, aSize * sizeof(a_T)); pipe.InitBuffer(inQueueB1, 1, bSize * sizeof(b_T)); pipe.InitBuffer(inQueueB2, 2, bSize * sizeof(b_T)); pipe.InitBuffer(outQueueCO1, 1, cSize * sizeof(dstCO1_T)); pipe.InitBuffer(deqQueue, 1, deqTensorLen * sizeof(uint64_t)); } __aicore__ inline void Process() { CopyIn(); SplitA(); SplitB(); Compute(); CopyOut(); } private: __aicore__ inline void CopyIn() { AscendC::LocalTensor<a_T> a1Local = inQueueA1.AllocTensor<a_T>(); AscendC::LocalTensor<b_T> b1Local = inQueueB1.AllocTensor<b_T>(); AscendC::LocalTensor<uint64_t> deqLocal = deqQueue.AllocTensor<uint64_t>(); AscendC::Nd2NzParams dataCopyA1Params; dataCopyA1Params.ndNum = 1; dataCopyA1Params.nValue = m; dataCopyA1Params.dValue = k; dataCopyA1Params.srcNdMatrixStride = 0; dataCopyA1Params.srcDValue = k; dataCopyA1Params.dstNzC0Stride = m; dataCopyA1Params.dstNzNStride = 1; dataCopyA1Params.dstNzMatrixStride = 0; AscendC::Nd2NzParams dataCopyB1Params; dataCopyB1Params.ndNum = 1; dataCopyB1Params.nValue = k; dataCopyB1Params.dValue = n; dataCopyB1Params.srcNdMatrixStride = 0; dataCopyB1Params.srcDValue = n; dataCopyB1Params.dstNzC0Stride = k; dataCopyB1Params.dstNzNStride = 1; dataCopyB1Params.dstNzMatrixStride = 0; // AscendC::DataCopy GM->L1:ND->大N小z AscendC::DataCopy(a1Local, aGM, dataCopyA1Params); AscendC::DataCopy(b1Local, bGM, dataCopyB1Params); AscendC::DataCopy(deqLocal, deqTensorGM, deqTensorLen); inQueueA1.EnQue(a1Local); inQueueB1.EnQue(b1Local); deqQueue.EnQue(deqLocal); } __aicore__ inline void SplitA() { AscendC::LocalTensor<a_T> a1Local = inQueueA1.DeQue<a_T>(); AscendC::LocalTensor<a_T> a2Local = inQueueA2.AllocTensor<a_T>(); AscendC::LoadData2dParams loadL0AParams; loadL0AParams.repeatTimes = mBlocks; loadL0AParams.srcStride = 1; loadL0AParams.dstGap = kBlocks - 1; loadL0AParams.ifTranspose = false; for (int i = 0; i < kBlocks; i++) { AscendC::LoadData(a2Local[i * AscendC::BLOCK_CUBE * (AscendC::ONE_BLK_SIZE / sizeof(a_T))], a1Local[i * m * (AscendC::ONE_BLK_SIZE / sizeof(a_T))], loadL0AParams); } inQueueA2.EnQue<a_T>(a2Local); inQueueA1.FreeTensor(a1Local); } __aicore__ inline void SplitB() { AscendC::LocalTensor<b_T> b1Local = inQueueB1.DeQue<b_T>(); AscendC::LocalTensor<b_T> b2Local = inQueueB2.AllocTensor<b_T>(); // load2d transpose L1->L0B AscendC::LoadData2dTransposeParams loadDataParams; loadDataParams.startIndex = 0; loadDataParams.srcStride = 1; loadDataParams.addrMode = 0; loadDataParams.repeatTimes = k * n / B8_SIZE; n_block = AscendC::ONE_BLK_SIZE; loadDataParams.dstGap = n / n_block - 1; loadDataParams.dstFracGap = 0; AscendC::LoadDataWithTranspose(b2Local, b1Local, loadDataParams); inQueueB1.FreeTensor(b1Local); inQueueB2.EnQue<b_T>(b2Local); } __aicore__ inline void Compute() { AscendC::LocalTensor<a_T> a2Local = inQueueA2.DeQue<a_T>(); AscendC::LocalTensor<b_T> b2Local = inQueueB2.DeQue<b_T>(); AscendC::LocalTensor<dstCO1_T> c1Local = outQueueCO1.AllocTensor<dstCO1_T>(); AscendC::MmadParams mmadParams; mmadParams.m = m; mmadParams.n = n; mmadParams.k = k; AscendC::Mmad(c1Local, a2Local, b2Local, mmadParams); // m*n outQueueCO1.EnQue<dstCO1_T>(c1Local); inQueueA2.FreeTensor(a2Local); inQueueB2.FreeTensor(b2Local); } __aicore__ inline void CopyOut() { AscendC::LocalTensor<dstCO1_T> c1Local = outQueueCO1.DeQue<dstCO1_T>(); AscendC::LocalTensor<uint64_t> deqTensorLocal = deqQueue.DeQue<uint64_t>(); AscendC::FixpipeParamsV220 fixpipeParams; fixpipeParams.nSize = n; fixpipeParams.mSize = m; fixpipeParams.srcStride = m; fixpipeParams.dstStride = n; fixpipeParams.ndNum = 1; fixpipeParams.srcNdStride = 4; fixpipeParams.dstNdStride = m*n; fixpipeParams.quantPre = QuantMode_t::VDEQF16; AscendC::Fixpipe(cGM, c1Local, deqTensorLocal, fixpipeParams); // CO1到GM可以进行NZ到ND的转换 outQueueCO1.FreeTensor(c1Local); deqQueue.FreeTensor(deqTensorLocal); } private: AscendC::TPipe pipe; AscendC::TQue<AscendC::QuePosition::A1, 1> inQueueA1; AscendC::TQue<AscendC::QuePosition::A2, 1> inQueueA2; AscendC::TQue<AscendC::QuePosition::B1, 1> inQueueB1; AscendC::TQue<AscendC::QuePosition::C1, 1> deqQueue; AscendC::TQue<AscendC::QuePosition::B2, 1> inQueueB2; AscendC::TQue<AscendC::QuePosition::CO1, 1> outQueueCO1; AscendC::GlobalTensor<a_T> aGM; AscendC::GlobalTensor<b_T> bGM; AscendC::GlobalTensor<c_T> cGM; AscendC::GlobalTensor<uint64_t> deqTensorGM; uint16_t m, k, n, n_mmad, startIndex, deqTensorLen; uint16_t B32_B16_SIZE = 16 * 16; uint16_t B8_SIZE = 32 * 32; uint8_t n_block = 16; bool L0Atranspose; uint8_t L0BtransposeMode; uint16_t aSize, bSize, cSize, b2Size, mBlocks, nBlocks, kBlocks; }; #define KERNEL_MATMUL(c_type, a_type, b_type, dstCO1_type, mIn, kIn, nIn) \ extern "C" __global__ __aicore__ void cube_matmul_operator( \ __gm__ uint8_t *a, __gm__ uint8_t *b, __gm__ uint8_t *c, __gm__ uint8_t *deq) \ { \ if (g_coreType == AscendC::AIV) { \ return; \ } \ KernelMatmul<c_type, a_type, b_type, dstCO1_type> op(mIn, kIn, nIn); \ op.Init(a, b, c, deq); \ op.Process(); \ } KERNEL_MATMUL(half, int8_t, int8_t, int32_t, 32, 32, 32);
示例结果:输入数据A矩阵: [[6 3 9 4 5 3 9 7 3 6 2 7 3 8 8 1 8 8 5 6 6 8 2 2 3 6 4 8 9 6 6 1] [2 5 7 2 4 2 5 2 4 6 4 8 5 7 1 4 3 1 8 6 4 6 9 1 8 2 9 5 3 7 7 8] [5 8 2 1 4 5 7 7 4 6 8 5 6 5 4 2 5 4 7 9 5 4 7 4 2 2 1 7 8 4 6 6] [8 2 4 7 6 9 7 7 4 5 6 7 6 6 5 3 7 6 7 4 5 4 1 9 6 7 8 9 4 9 5 5] [4 9 4 2 7 8 3 4 1 5 3 8 8 5 5 8 3 8 5 3 9 4 5 4 2 4 3 8 9 8 4 3] [1 3 8 3 1 9 9 5 5 6 3 2 3 4 3 3 5 9 6 7 1 3 4 2 8 5 9 1 9 5 8 9] [3 3 1 3 5 2 7 8 8 9 6 9 3 6 5 5 2 3 2 3 5 1 6 1 7 8 7 2 2 7 8 1] [4 4 6 4 6 5 1 2 7 8 3 2 9 9 7 7 7 1 2 7 2 1 5 2 1 3 2 1 3 3 2 9] [4 6 3 5 8 4 1 1 2 5 8 8 8 3 9 6 5 6 7 9 2 1 9 3 2 5 4 1 7 5 3 9] [7 2 3 4 9 5 6 3 4 5 4 7 4 1 9 4 2 1 7 4 9 2 4 5 4 5 8 7 2 2 8 3] [5 7 6 2 9 4 7 1 8 6 2 1 6 5 5 6 3 8 1 5 2 1 8 3 1 9 3 3 5 2 2 5] [4 7 5 9 9 6 7 3 1 9 2 6 5 2 6 7 1 7 6 9 3 7 6 1 3 9 2 4 1 9 4 8] [2 4 3 1 1 2 2 7 2 3 7 9 8 8 3 4 1 2 9 2 9 4 4 8 5 7 7 3 9 9 5 3] [3 1 1 6 1 8 3 3 6 3 4 4 3 8 2 1 1 1 6 5 8 8 5 8 5 1 2 2 1 3 7 4] [4 2 8 4 4 1 9 6 9 9 5 4 3 1 3 8 1 2 8 2 5 8 9 3 2 5 9 7 7 4 2 1] [2 6 7 1 3 9 9 9 6 4 5 8 1 3 7 3 8 7 3 4 8 6 9 6 8 9 4 4 7 6 1 4] [2 8 2 1 2 6 2 8 5 9 9 8 6 4 4 1 4 1 4 4 4 7 5 9 9 8 9 1 8 4 7 3] [3 6 2 5 1 2 9 2 6 7 4 5 9 6 5 9 7 9 5 5 6 7 4 7 7 6 3 6 5 2 8 3] [1 7 3 2 4 8 1 7 3 4 1 6 1 4 4 1 6 7 9 3 9 2 2 2 2 8 1 1 6 3 6 1] [4 3 9 5 2 2 1 8 5 8 9 2 4 3 2 1 8 6 6 2 9 2 9 3 9 5 3 7 9 7 6 2] [9 4 8 1 3 7 9 5 2 4 9 9 6 9 6 4 6 3 3 9 6 8 1 5 5 1 6 5 1 9 3 9] [2 5 2 1 8 9 9 8 1 6 1 1 9 8 3 5 6 4 2 1 3 7 8 9 6 6 1 9 1 7 6 8] [4 7 6 6 2 2 1 8 7 1 1 2 1 1 9 8 9 4 9 5 7 8 9 9 5 1 6 8 9 6 7 5] [1 1 6 9 9 3 7 6 5 6 5 1 5 5 3 7 6 7 4 8 8 2 2 5 7 8 8 2 9 1 5 1] [5 4 6 8 8 3 7 7 5 7 8 7 4 8 2 9 4 8 1 3 8 5 3 7 3 7 1 9 1 5 4 7] [6 3 1 2 8 3 2 6 8 2 8 4 1 9 4 7 5 1 7 5 5 1 1 1 2 8 1 7 9 8 5 4] [2 8 5 1 3 4 9 8 6 9 6 2 4 2 2 7 8 2 1 3 7 1 4 6 4 6 3 3 1 6 8 3] [5 1 5 5 9 7 9 2 1 4 7 8 1 9 8 1 2 4 3 9 9 6 7 9 1 5 1 9 2 5 6 9] [1 9 9 6 5 7 9 5 4 1 2 8 3 8 1 9 6 1 7 9 3 2 2 4 7 9 9 4 7 1 5 8] [3 2 2 5 9 3 6 9 2 4 4 8 4 2 6 1 2 8 8 8 9 7 7 1 9 6 5 8 3 3 3 4] [9 1 6 1 3 7 8 1 2 6 5 9 4 4 7 2 3 9 8 7 8 2 6 4 5 6 5 4 9 6 1 9] [4 3 2 7 8 1 7 2 9 7 7 4 2 8 2 5 6 9 5 1 3 9 8 2 4 8 4 7 4 1 3 7]] 输入数据B矩阵: [[3 5 9 6 2 9 3 6 5 9 5 5 3 8 5 2 5 1 5 7 5 4 2 2 4 8 1 1 3 3 7 2] [6 7 4 6 1 4 8 3 9 2 2 3 4 6 5 3 4 8 2 6 4 8 6 7 3 8 6 7 3 8 1 1] [6 7 8 6 1 9 9 3 9 9 2 1 3 3 3 3 7 2 4 7 5 8 9 2 1 7 9 6 8 7 1 3] [3 3 9 2 3 9 4 1 8 2 5 1 2 6 5 5 6 4 8 8 7 5 9 6 7 6 8 8 2 6 1 2] [4 2 3 8 6 1 1 1 7 9 5 2 2 5 7 6 4 5 9 5 6 8 1 2 1 9 2 7 8 6 6 1] [4 8 6 6 3 1 7 8 7 3 2 9 8 6 9 8 3 2 5 5 7 9 7 7 4 8 3 5 2 7 1 2] [3 8 2 8 9 5 1 5 7 4 1 3 4 1 4 6 9 5 2 2 4 6 3 3 7 1 9 6 8 6 4 7] [2 3 8 1 5 9 8 4 5 4 6 5 4 5 3 2 3 5 4 2 1 2 9 2 3 8 9 8 8 1 2 7] [1 4 5 1 3 8 2 5 9 9 5 5 5 6 4 2 7 6 7 7 6 9 1 3 8 1 9 8 8 5 1 6] [5 3 8 9 8 2 6 6 1 3 2 1 2 9 3 9 1 1 4 9 8 6 6 5 6 8 4 2 2 7 2 1] [8 1 3 5 8 7 5 7 4 6 7 4 8 2 2 3 5 8 6 8 1 8 6 8 3 9 1 1 3 8 3 2] [7 7 5 1 5 4 6 1 1 6 8 8 1 7 7 2 1 7 7 7 7 6 1 7 3 3 8 9 3 8 9 8] [4 9 5 6 9 6 8 9 1 1 6 5 1 4 3 5 4 1 8 9 6 5 5 7 8 9 8 2 7 5 5 3] [9 8 4 9 5 4 7 5 7 6 9 8 5 7 2 9 6 6 5 1 4 5 9 6 7 5 5 2 3 7 6 5] [5 2 5 7 9 2 2 3 2 3 1 4 6 5 3 1 5 1 9 3 2 4 1 6 7 7 4 9 8 8 6 1] [3 7 5 6 7 8 2 2 8 7 6 1 3 5 3 2 7 6 7 8 6 5 2 2 8 2 2 6 6 4 9 6] [4 8 4 7 6 4 1 5 1 7 2 4 1 1 5 5 3 5 2 2 7 5 4 7 5 8 2 4 6 2 8 9] [9 2 7 4 1 7 4 4 7 1 9 7 4 5 3 8 7 8 8 4 1 9 9 8 4 9 3 1 1 8 6 3] [4 9 2 7 3 9 5 2 6 8 8 7 1 5 6 1 9 4 1 6 1 6 2 1 3 5 2 6 6 8 1 9] [8 3 9 4 9 7 7 4 2 8 4 1 7 9 3 9 1 3 8 7 6 1 4 9 1 6 8 7 6 3 2 2] [2 3 4 5 4 9 9 3 4 4 7 3 8 7 9 7 7 5 8 5 8 4 1 8 1 9 5 8 8 3 9 5] [7 7 5 6 6 1 4 7 9 7 6 2 3 5 7 1 3 5 9 2 2 4 6 9 4 5 9 7 2 3 8 3] [2 9 2 4 1 4 7 2 5 4 8 8 2 3 3 3 1 3 5 9 5 8 3 8 6 8 4 1 1 6 1 7] [7 1 8 5 2 6 6 6 7 1 7 4 2 1 5 9 6 4 2 8 4 3 2 5 9 1 3 9 1 9 3 9] [9 4 4 9 4 9 4 5 4 1 3 2 6 5 6 1 8 2 4 1 7 5 9 3 5 7 9 3 9 4 1 4] [1 6 2 1 7 1 5 2 8 8 6 4 4 2 5 2 5 8 1 2 9 3 1 1 8 6 9 4 2 2 1 8] [9 1 8 3 8 7 1 6 2 3 8 1 4 8 6 7 4 8 5 9 3 7 4 1 3 8 4 3 3 3 2 4] [9 4 5 6 2 2 3 7 2 2 3 3 2 8 5 4 5 5 5 5 1 5 8 4 4 1 1 3 8 5 3 8] [6 3 6 7 9 9 4 5 9 2 6 6 4 9 9 2 8 9 4 7 4 7 4 4 6 8 9 6 2 7 3 6] [9 1 5 8 8 8 5 9 6 8 4 9 4 2 3 6 2 2 4 8 2 6 6 4 6 7 6 9 5 8 5 9] [5 5 5 9 2 4 6 3 1 5 2 2 8 6 3 2 6 2 7 8 7 9 6 2 6 6 1 5 1 3 4 7] [6 6 9 1 2 3 4 1 1 5 3 2 3 4 5 5 3 8 6 6 9 1 5 9 2 2 9 4 4 6 2 2]] 输入数据量化Tensor: [1065353216 1073741824 1065353216 1073741824 1065353216 1065353216 1065353216 1073741824 1073741824 1073741824 1065353216 1065353216 1065353216 1065353216 1065353216 1073741824 1073741824 1065353216 1073741824 1065353216 1073741824 1073741824 1065353216 1065353216 1073741824 1065353216 1073741824 1073741824 1065353216 1073741824 1065353216 1073741824] 输出数据C矩阵: [[ 943. 1676. 932. 1962. 893. 941. 817. 1528. 1778. 1740. 823. 715. 659. 915. 818. 1500. 1710. 794. 1824. 890. 1558. 1938. 846. 827. 1596. 1066. 1916. 1842. 822. 1860. 724. 1702.] [ 889. 1638. 814. 1730. 757. 863. 772. 1326. 1454. 1592. 780. 620. 582. 821. 720. 1326. 1430. 715. 1632. 930. 1534. 1790. 751. 762. 1380. 921. 1736. 1546. 721. 1712. 564. 1524.] [ 855. 1614. 847. 1774. 805. 873. 817. 1442. 1548. 1544. 776. 690. 638. 849. 744. 1416. 1486. 755. 1668. 927. 1472. 1798. 750. 853. 1456. 984. 1682. 1630. 731. 1800. 596. 1530.] [1033. 1746. 1044. 2034. 940. 1044. 873. 1764. 1860. 1816. 931. 802. 717. 951. 910. 1742. 1832. 857. 1934. 1053. 1770. 2082. 904. 883. 1818. 1126. 1934. 1972. 867. 2074. 729. 1890.] [ 902. 1650. 872. 1874. 821. 897. 850. 1482. 1736. 1530. 846. 746. 632. 897. 830. 1496. 1582. 793. 1814. 976. 1564. 1954. 770. 851. 1546. 1058. 1686. 1766. 749. 1930. 715. 1588.] [ 886. 1578. 900. 1740. 799. 913. 756. 1410. 1630. 1492. 737. 643. 666. 819. 749. 1458. 1612. 762. 1596. 893. 1574. 1878. 832. 759. 1494. 979. 1866. 1572. 703. 1750. 503. 1498.] [ 753. 1364. 754. 1576. 802. 818. 702. 1262. 1416. 1494. 746. 617. 612. 775. 655. 1254. 1380. 690. 1578. 845. 1496. 1734. 663. 659. 1500. 908. 1638. 1544. 693. 1566. 569. 1492.] [ 677. 1428. 767. 1478. 708. 704. 662. 1154. 1298. 1428. 627. 533. 502. 709. 580. 1288. 1192. 585. 1526. 810. 1478. 1478. 617. 716. 1342. 833. 1472. 1348. 647. 1508. 521. 1106.] [ 851. 1560. 858. 1662. 837. 854. 766. 1264. 1496. 1588. 813. 677. 589. 821. 730. 1388. 1402. 758. 1792. 994. 1588. 1796. 673. 863. 1472. 1029. 1650. 1616. 687. 1884. 613. 1378.] [ 751. 1388. 793. 1644. 755. 802. 683. 1236. 1374. 1494. 723. 569. 600. 811. 750. 1276. 1482. 652. 1674. 888. 1500. 1702. 591. 673. 1378. 906. 1442. 1632. 739. 1614. 605. 1420.] [ 683. 1436. 740. 1504. 696. 720. 652. 1160. 1588. 1438. 681. 568. 526. 711. 630. 1306. 1376. 683. 1508. 816. 1456. 1684. 607. 682. 1422. 866. 1542. 1366. 643. 1590. 511. 1224.] [ 873. 1678. 919. 1798. 854. 850. 814. 1350. 1750. 1726. 784. 651. 619. 864. 775. 1522. 1492. 748. 1870. 977. 1714. 1850. 789. 857. 1558. 1029. 1886. 1812. 750. 1896. 632. 1446.] [ 854. 1464. 787. 1644. 810. 922. 822. 1400. 1542. 1450. 872. 707. 599. 785. 745. 1294. 1520. 757. 1536. 902. 1398. 1682. 690. 730. 1500. 946. 1704. 1658. 676. 1736. 611. 1680.] [ 657. 1252. 676. 1350. 557. 690. 661. 1132. 1282. 1196. 651. 539. 538. 654. 614. 1168. 1210. 530. 1388. 705. 1246. 1370. 597. 674. 1216. 711. 1338. 1362. 524. 1372. 470. 1212.] [ 761. 1524. 814. 1636. 805. 906. 706. 1358. 1718. 1606. 797. 590. 549. 813. 730. 1230. 1568. 737. 1604. 945. 1396. 1830. 676. 670. 1516. 895. 1726. 1626. 744. 1676. 560. 1574.] [ 912. 1756. 910. 1832. 874. 961. 873. 1544. 1906. 1696. 859. 785. 715. 847. 875. 1508. 1694. 861. 1762. 916. 1704. 2014. 818. 901. 1670. 1089. 2064. 1926. 836. 1946. 666. 1806.] [ 903. 1526. 879. 1748. 865. 887. 848. 1536. 1604. 1480. 834. 677. 672. 853. 800. 1386. 1490. 792. 1634. 954. 1610. 1864. 768. 811. 1610. 1047. 1858. 1710. 677. 1794. 566. 1592.] [ 908. 1756. 893. 1928. 866. 944. 805. 1522. 1728. 1538. 847. 664. 653. 868. 779. 1504. 1772. 805. 1832. 954. 1686. 1930. 801. 870. 1814. 986. 1836. 1724. 773. 1860. 711. 1700.] [ 610. 1272. 634. 1334. 578. 681. 674. 988. 1342. 1236. 636. 585. 520. 666. 652. 1082. 1238. 615. 1248. 652. 1246. 1472. 570. 612. 1110. 836. 1324. 1412. 551. 1374. 483. 1278.] [ 853. 1486. 856. 1790. 754. 997. 838. 1456. 1616. 1528. 807. 674. 638. 819. 749. 1328. 1606. 731. 1614. 937. 1520. 1904. 841. 777. 1492. 1082. 1710. 1552. 756. 1740. 560. 1640.] [1024. 1736. 989. 1946. 916. 966. 862. 1676. 1646. 1832. 833. 722. 712. 886. 804. 1638. 1594. 783. 1904. 970. 1644. 1860. 852. 933. 1534. 1041. 1912. 1826. 846. 1946. 753. 1588.] [ 853. 1726. 833. 1888. 777. 757. 798. 1534. 1634. 1460. 752. 692. 594. 749. 748. 1548. 1490. 705. 1644. 850. 1588. 1772. 818. 816. 1664. 945. 1706. 1618. 753. 1764. 625. 1636.] [ 903. 1646. 959. 1848. 781. 1035. 813. 1446. 1828. 1662. 849. 684. 647. 892. 839. 1332. 1736. 803. 1822. 1004. 1540. 1914. 792. 840. 1662. 1018. 1802. 1992. 818. 1854. 663. 1820.] [ 827. 1442. 887. 1760. 882. 972. 749. 1342. 1744. 1552. 826. 570. 655. 850. 779. 1530. 1724. 791. 1758. 908. 1654. 1836. 766. 737. 1568. 1034. 1812. 1700. 781. 1676. 603. 1512.] [ 915. 1642. 953. 1814. 825. 944. 842. 1466. 1836. 1736. 883. 674. 656. 868. 787. 1622. 1698. 852. 1922. 973. 1722. 1918. 853. 875. 1672. 999. 1836. 1810. 809. 1922. 733. 1656.] [ 742. 1342. 725. 1580. 765. 819. 656. 1236. 1544. 1652. 739. 639. 592. 770. 681. 1164. 1454. 732. 1506. 794. 1358. 1612. 621. 641. 1382. 857. 1456. 1548. 704. 1552. 585. 1500.] [ 699. 1408. 751. 1612. 729. 795. 720. 1298. 1438. 1414. 632. 540. 590. 674. 633. 1310. 1380. 656. 1392. 826. 1484. 1658. 670. 675. 1440. 871. 1522. 1530. 697. 1508. 541. 1466.] [ 932. 1604. 911. 1844. 817. 824. 835. 1416. 1644. 1710. 826. 701. 693. 857. 806. 1668. 1560. 768. 1910. 937. 1660. 1810. 759. 924. 1522. 963. 1734. 1828. 760. 1958. 697. 1582.] [ 909. 1844. 923. 1772. 851. 962. 825. 1330. 1844. 1736. 823. 639. 662. 889. 841. 1492. 1742. 884. 1674. 940. 1800. 1892. 809. 782. 1574. 966. 2034. 1866. 814. 1826. 592. 1686.] [ 861. 1508. 839. 1670. 806. 884. 777. 1308. 1542. 1538. 838. 650. 627. 865. 799. 1362. 1530. 753. 1824. 848. 1496. 1744. 755. 811. 1362. 1018. 1798. 1700. 809. 1690. 628. 1524.] [ 916. 1632. 918. 1792. 847. 948. 807. 1450. 1622. 1644. 848. 752. 655. 883. 830. 1530. 1636. 784. 1750. 959. 1636. 1852. 725. 860. 1498. 1032. 1818. 1660. 752. 1950. 662. 1574.] [ 822. 1602. 807. 1662. 757. 812. 678. 1306. 1734. 1624. 840. 633. 568. 804. 737. 1366. 1586. 830. 1734. 860. 1544. 1862. 747. 801. 1578. 921. 1696. 1490. 689. 1740. 622. 1506.]]