DataCopy
功能说明
数据搬运接口,支持的数据传输通路如下:
GM->A1
GM->B1
CO1->CO2
CO2->GM
GM->VECIN
VECIN->VECOUT
VECOUT->GM
定义原型
普通数据搬运接口,适用于连续和不连续数据搬运:
- 通路:GM->VECIN, GM->A1, GM->B1
template <typename T> void DataCopy(const LocalTensor<T>& dstLocal, const GlobalTensor<T>& srcGlobal, const DataCopyParams& intriParams);
- 通路:VECIN->VECOUT
template <typename T> void DataCopy(const LocalTensor<T>& dstLocal, const LocalTensor <T>& srcLocal, const DataCopyParams& intriParams);
- 通路:VECOUT->GM, CO2->GM
template <typename T> void DataCopy(const GlobalTensor <T>& dstGlobal, const LocalTensor <T>& srcLocal, const DataCopyParams& intriParams);
普通数据搬运接口,适用于连续数据搬运:
- 通路:GM->VECIN, GM->A1, GM->B1
template <typename T> void DataCopy(const LocalTensor<T>& dstLocal, const GlobalTensor<T>& srcGlobal, const uint32_t calCount);
- 通路:VECIN->VECOUT
template <typename T> void DataCopy(const LocalTensor<T>& dstLocal, const LocalTensor <T>& srcLocal, const uint32_t calCount);
- 通路:VECOUT->GM, CO2->GM
template <typename T> void DataCopy(const GlobalTensor <T>& dstGlobal, const LocalTensor <T>& srcLocal, const uint32_t calCount);
增强数据搬运接口,相比于普通数据搬运接口,搬运时增加了随路计算:
- 通路:GM->VECIN, GM->A1, GM->B1
template <typename T> __aicore__ inline void DataCopy(const LocalTensor<T>& dstLocal, const GlobalTensor<T>& srcGlobal, const DataCopyParams& intriParams, const DataCopyEnhancedParams& enhancedParams)
- 通路:VECIN->VECOUT, CO1->CO2
template <typename T> __aicore__ inline void DataCopy(const LocalTensor<T>& dstLocal, const LocalTensor<T>& srcLocal, const DataCopyParams& intriParams, const DataCopyEnhancedParams& enhancedParams)
- 通路:VECOUT->GM, CO2->GM
template <typename T> __aicore__ inline void DataCopy(const GlobalTensor<T>& dstGlobal, const LocalTensor<T>& srcLocal, const DataCopyParams& intriParams, const DataCopyEnhancedParams& enhancedParams)
切片数据搬运,主要适用于非连续vector数据搬运:
- 通路:GM->VECIN
template <typename T> void DataCopy(const LocalTensor<T> &dstLocal, const GlobalTensor<T> &srcGlobal, const SliceInfo dstSliceInfo[], const SliceInfo srcSliceInfo[], const uint32_t dimValue = 1)
- 通路:VECOUT->GM, CO2->GM
template <typename T> void DataCopy(const GlobalTensor<T> &dstGlobal, const LocalTensor<T> &srcLocal, const SliceInfo dstSliceInfo[], const SliceInfo srcSliceInfo[], const uint32_t dimValue = 1)
随路格式转换数据搬运,适用于cube数据搬运,在搬运时进行格式转换:
- 通路:GM->A1, GM->B1(ND2NZ)
template <typename T> void DataCopy(const LocalTensor<T>& dstLocal, const GlobalTensor<T>& srcGlobal, const Nd2NzParams& intriParams);
注意:针对如下产品型号,使用该接口时需要预留8K的Unified Buffer空间,作为接口的临时数据存放区。
- Atlas 训练系列产品
- Atlas推理系列产品(Ascend 310P处理器)AI Core
- Atlas A2训练系列产品/Atlas 800I A2推理产品
- 通路:VECOUT->GM(NZ2ND)
template <typename T> void DataCopy(const GlobalTensor <T>& dstGlobal, const LocalTensor <T>& srcLocal, const Nz2NdParamsFull &intriParams);
参数说明(普通数据搬运)
参数名称 |
输入/输出 |
含义 |
---|---|---|
dstLocal, dstGlobal |
输出 |
目的操作数,类型为LocalTensor或GlobalTensor。支持的数据类型为:half/int16_t/uint16_t/float/int32_t/uint32_t/int8_t/uint8_t。 |
srcLocal, srcGlobal |
输入 |
源操作数,类型为LocalTensor或GlobalTensor。支持的数据类型为:half/int16_t/uint16_t/float/int32_t/uint32_t/int8_t/uint8_t。 |
intriParams |
输入 |
搬运参数,DataCopyParams类型,DataCopyParams结构定义请参考表2。 |
calCount |
输入 |
参与搬运的元素个数。 |
参数名称 |
含义 |
---|---|
blockCount |
指定该指令包含的连续传输数据块个数,取值范围:blockCount∈[1, 4095]。 |
blockLen |
指定该指令每个连续传输数据块长度,单位为datablock(32Bytes)。取值范围:blockLen∈[1, 65535]。 |
srcStride |
源操作数,相邻连续数据块的间隔(前面一个数据块的尾与后面数据块的头的间隔),单位为datablock(32Bytes)。 |
dstStride |
目的操作数,相邻连续数据块间的间隔(前面一个数据块的尾与后面数据块的头的间隔),单位为datablock(32Bytes)。 |
下面的样例呈现了DataCopyParams结构体参数的使用方法,样例中完成了2个连续传输数据块的搬运,每个数据块含有8个block,源操作数相邻数据块之间无间隔,目的操作数相邻数据块尾与头之间间隔1个block。
参数说明(增强数据搬运)
参数名称 |
输入/输出 |
含义 |
---|---|---|
dstLocal, dstGlobal |
输出 |
目的操作数,类型为LocalTensor或GlobalTensor。支持的数据类型为:half/int16_t/uint16_t/float/int32_t/uint32_t/int8_t/uint8_t。 |
srcLocal, srcGlobal |
输入 |
源操作数,类型为LocalTensor或GlobalTensor。支持的数据类型为:half/int16_t/uint16_t/float/int32_t/uint32_t/int8_t/uint8_t。 |
intriParams |
输入 |
搬运参数。DataCopyParams类型,DataCopyParams结构定义请参考表2。 |
enhancedParams |
输入 |
增强信息参数。 DataCopyEnhancedParams类型, DataCopyEnhancedParams结构定义如下: struct DataCopyEnhancedParams { BlockMode blockMode = BlockMode::BLOCK_MODE_NORMAL; DeqScale deqScale = DeqScale::DEQ_NONE; uint64_t deqValue = 0; uint8_t sidStoreMode = 0; bool isRelu = false; pad_t padMode = pad_t::PAD_NONE; uint64_t padValue = 0; uint64_t deqTensorAddr = 0; }; 具体参数说明请参考表4。 |
参数名称 |
含义 |
---|---|
blockMode |
数据搬移基本分形,传入enum BlockMode,支持以下 5 种配置:
|
deqScale |
随路精度转换辅助参数,即量化模式,仅用于CO1->CO2通路。支持如表5所示情况。 |
deqValue |
随路精度转换大小。 |
deqTensorAddr |
该参数为预留参数,开发者无需关注。 |
sidStoreMode |
用于配置存储模式或sid。在 CO1->CO2 通路、并且量化模式为 deq8/vdeq8 时,dst一侧的每2个block(对应src一侧的1*16分形)会合成1个16B,该参数在此时功能表现为storeMode,用来指定该 16B 的存储模式,支持以下配置:
在CO1->CO2以外的通路,该配置表现为sid。 |
isRelu |
仅用于 CO1->CO2 通路,配置是否可以随路做线性整流操作。 |
padMode |
仅用于 GM->A1/GM->B1通路,用于随路加pad补齐,当前暂不支持。 |
量化名 |
src.dtype |
dst.dtype |
---|---|---|
DEQ |
int32_t |
half |
DEQ |
half |
half |
VDEQ |
int32_t |
half |
DEQ8 |
int32_t |
int8_t |
DEQ8 |
int32_t |
uint8_t |
VDEQ8 |
int32_t |
int8_t |
VDEQ8 |
int32_t |
uint8_t |
DEQ16 |
int32_t |
half |
DEQ16 |
int32_t |
int16_t |
VDEQ16 |
int32_t |
half |
VDEQ16 |
int32_t |
int16_t |
src 通路 |
dst 通路 |
数据类型 |
blockCount 单位 |
srcStride单位 |
dstStride单位 |
---|---|---|---|---|---|
GM |
A1 |
b8, b16, b32 |
32B |
32B |
32B |
GM |
B1 |
b8, b16, b32 |
32B |
32B |
32B |
GM |
VECIN |
b8, b16, b32 |
32B |
32B |
32B |
VECOUT |
GM |
b8, b16, b32 |
32B |
32B |
32B |
VECIN |
VECOUT |
b8, b16, b32 |
32B |
32B |
32B |
src 通路 |
dst 通路 |
数据类型 |
blockCount 单位 |
srcStride单位 |
dstStride单位 |
---|---|---|---|---|---|
CO1 |
CO2 |
b16 |
512B |
512B |
32B |
CO1 |
CO2 |
b32 |
1024B |
1024B |
32B |
src 通路 |
dst 通路 |
数据类型 |
blockCount 单位 |
srcStride 单位 |
dstStride 单位 |
---|---|---|---|---|---|
CO1 |
CO2 |
b16 |
32B |
512B |
32B |
CO1 |
CO2 |
b32 |
64B |
1024B |
32B |
src 通路 |
dst 通路 |
src 数据类型 |
dst 数据类型 |
---|---|---|---|
CO1 |
CO2 |
float |
half |
CO1 |
CO2 |
int32_t |
half |
CO1 |
CO2 |
int32_t |
int16_t |
CO1 |
CO2 |
int32_t |
int8_t |
CO1 |
CO2 |
int32_t |
uint8_t |
参数说明(切片数据搬运)
参数名称 |
输入/输出 |
含义 |
---|---|---|
dstLocal, dstGlobal |
输出 |
目的操作数,类型为LocalTensor或GlobalTensor。支持的数据类型为:half/int16_t/uint16_t/float/int32_t/uint32_t/int8_t/uint8_t。 |
srcLocal, srcGlobal |
输入 |
源操作数,类型为LocalTensor或GlobalTensor。支持的数据类型为:half/int16_t/uint16_t/float/int32_t/uint32_t/int8_t/uint8_t。 |
srcSliceInfo/dstSliceInfo |
输入 |
目的操作数/源操作数切片信息,SliceInfo类型,SliceInfo具体参数请参考表11。 |
dimValue |
输入 |
操作数维度信息,默认值为1。 |
参数说明(随路格式转换)
参数名称 |
含义 |
---|---|
ndNum |
传输nd矩阵的数目,取值范围:ndNum∈[0, 4095]。 |
nValue |
nd矩阵的行数,取值范围:nValue∈[0, 16384]。 |
dValue |
nd矩阵的列数,取值范围:dValue∈[0, 65535]。 |
srcNdMatrixStride |
源操作数相邻nd矩阵起始地址间的偏移,取值范围:srcNdMatrixStride∈[0, 65535],单位:element。 |
srcDValue |
源操作数同一nd矩阵的相邻行起始地址间的偏移,取值范围:srcDValue∈[1, 65535],单位:element。 |
dstNzC0Stride |
ND转换到NZ格式后,源操作数中的一行会转换为目的操作数的多行。dstNzC0Stride表示,目的nz矩阵中,来自源操作数同一行的多行数据相邻行起始地址间的偏移,取值范围:dstNzC0Stride∈[1, 16384],单位:C0_SIZE(32B)。 |
dstNzNStride |
目的nz矩阵中,Z型矩阵相邻行起始地址之间的偏移。取值范围:dstNzNStride∈[1, 16384],单位:C0_SIZE(32B)。 |
dstNzMatrixStride |
目的nz矩阵中,相邻nz矩阵起始地址间的偏移,取值范围:dstNzMatrixStride∈[1, 65535],单位:element。 |
ND2NZ转换示意图如下,为了方便展示和理解,这里我们假设分形矩阵的大小是2x2,ND矩阵的大小是4x4,请注意这与实际的情况并不相符。样例中参数设置值和解释说明如下:
- ndNum = 1,表示传输nd矩阵的数目为1。nValue=4,矩阵的行数,也就是矩阵的高度为4。dValue=4,矩阵的列数,也就是矩阵的宽度为4。
- srcNdMatrixStride = 0,当前只有一个nd矩阵,不存在相邻nd矩阵起始地址间的偏移,设置为0。同理,dstNzMatrixStride =0。
- srcDValue= dValue, 源操作数同一nd矩阵的相邻行起始地址间的偏移,也就是图中addr1和addr2之间的偏移。
- dstNzC0Stride = nValue *C0_SIZE/C0_SIZE。ND转换到NZ格式后,源操作数中的一行会转换为目的操作数的多行,也就是图中的line_src会转换为line_dst1和line_dst2。多行数据起始地址之间的偏移就是addr3和addr4之间的偏移,偏移为nValue *C0_SIZE,因为该参数的单位为C0_SIZE,所以,dstNzC0Stride = nValue *C0_SIZE/C0_SIZE。
- dstNzNStride=C0_SIZE/C0_SIZE,即图中addr3和addr5之间的偏移C0_SIZE,因为该参数的单位为C0_SIZE,所以,dstNzNStride=C0_SIZE/C0_SIZE。
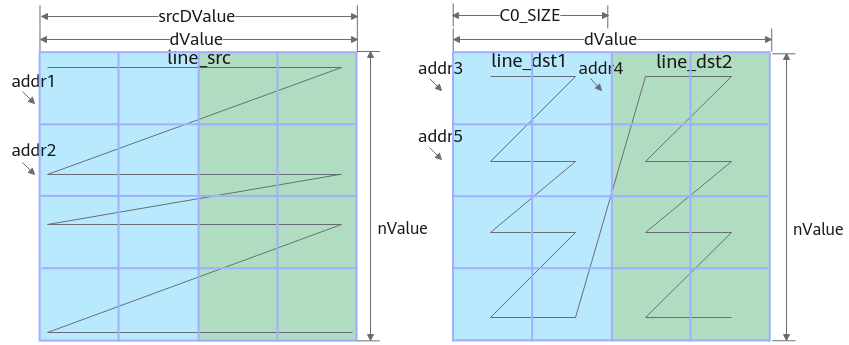
参数名称 |
含义 |
---|---|
ndNum |
传输nz矩阵的数目,取值范围:ndNum∈[0, 4095]。 |
nValue |
nz矩阵的行数,取值范围:nValue∈[1, 8192]。 |
dValue |
nz矩阵的列数,取值范围:dValue∈[1, 8192]。 |
srcNdMatrixStride |
源相邻nz矩阵的偏移(头与头),取值范围:srcNdMatrixStride∈[1, 512],单位fractal_size(16*16)。 |
srcNStride |
源同一nz矩阵的相邻z排布的偏移(头与头),取值范围:srcNStride∈[0, 4096],单位C0_size(16)。 |
dstDStride |
目的nd矩阵的相邻行的偏移(头与头),取值范围:dstDStride∈[1, 65535],单位:element。 |
dstNdMatrixStride |
目的nd矩阵中,来自源相邻nz矩阵的偏移(头与头),取值范围:dstNdMatrixStride∈[1, 65535],单位:element。 |
支持的型号
Atlas 训练系列产品
Atlas推理系列产品(Ascend 310P处理器)AI Core
Atlas推理系列产品(Ascend 310P处理器)Vector Core(当前仅支持普通数据搬运的GM -> VECIN、VECOUT->GM通路)
Atlas A2训练系列产品/Atlas 800I A2推理产品
Atlas 200/500 A2推理产品(当前仅支持普通数据搬运的GM -> VECIN、VECOUT->GM通路)
注意事项
- 增强搬运主要分为CO1->CO2及其他情况两种。
- DataCopy的搬运量要求为32byte的倍数,因此使用普通数据搬运接口(适用于连续数据搬运,包含calCount参数)时,calCount * sizeof(T)需要32byte对齐,若不对齐,搬运量将对32byte做向下取整。
- 配置relu与deqScale的某bit配置冲突时,以relu为准。
- 切片数据搬运中的横向burstLen大小设置,需要用户自己通过计算:横向切片元素个数* sizeof(T)/32byte。横向切片元素个数* sizeof(T)的大小必须32byte的倍数。
- 切片数据搬运中的SliceInfo结构体数组大小和dimValue需要保持一致,并且不超过8。
- 切片数据搬运中的srcSliceInfo数组大小的和dstSliceInfo的大小需要保持一致,两者的结构体中的burstLen需要相等(srcSliceInfo[i].burstLen = dstSliceInfo[i].burstLen)。
- 切片数据搬运对参数有一定要求,建议使用者参考调用示例,并在CPU上仿真结果无误后,再到NPU侧执行。
- 如果 CO1->CO2有随路精度转换,通路为 UB 的操作数的 blockCount 单位需要减半。
- 随路格式转换nd2nz只支持GM->A1,GM->B1; nz2nd只支持VECOUT->GM。
- 硬件在执行数据搬运时会以block作为基本单位,而 1 block = 32 Byte,故使用者可以尝试通过每次指令处理32Byte整数倍大小的数据来提高指令的执行效率。
返回值
无
调用示例
- 普通数据搬运接口
#include "kernel_operator.h" namespace AscendC { class KernelDataCopy { public: __aicore__ inline KernelDataCopy() {} __aicore__ inline void Init(__gm__ uint8_t* src0Gm, __gm__ uint8_t* src1Gm, __gm__ uint8_t* dstGm) { src0Global.SetGlobalBuffer((__gm__ half*)src0Gm); src1Global.SetGlobalBuffer((__gm__ half*)src1Gm); dstGlobal.SetGlobalBuffer((__gm__ half*)dstGm); pipe.InitBuffer(inQueueSrc0, 1, 512 * sizeof(half)); pipe.InitBuffer(inQueueSrc1, 1, 512 * sizeof(half)); pipe.InitBuffer(outQueueDst, 1, 512 * sizeof(half)); } __aicore__ inline void Process() { CopyIn(); Compute(); CopyOut(); } private: __aicore__ inline void CopyIn() { LocalTensor<half> src0Local = inQueueSrc0.AllocTensor<half>(); LocalTensor<half> src1Local = inQueueSrc1.AllocTensor<half>(); DataCopy(src0Local, src0Global, 512); DataCopy(src1Local, src1Global, 512); inQueueSrc0.EnQue(src0Local); inQueueSrc1.EnQue(src1Local); } __aicore__ inline void Compute() { LocalTensor<half> src0Local = inQueueSrc0.DeQue<half>(); LocalTensor<half> src1Local = inQueueSrc1.DeQue<half>(); LocalTensor<half> dstLocal = outQueueDst.AllocTensor<half>(); Add(dstLocal, src0Local, src1Local, 512); outQueueDst.EnQue<half>(dstLocal); inQueueSrc0.FreeTensor(src0Local); inQueueSrc1.FreeTensor(src1Local); } __aicore__ inline void CopyOut() { LocalTensor<half> dstLocal = outQueueDst.DeQue<half>(); DataCopy(dstGlobal, dstLocal, 512); outQueueDst.FreeTensor(dstLocal); } private: TPipe pipe; TQue<QuePosition::VECIN, 1> inQueueSrc0, inQueueSrc1; TQue<QuePosition::VECOUT, 1> outQueueDst; GlobalTensor<half> src0Global, src1Global, dstGlobal; }; } // namespace AscendC extern "C" __global__ __aicore__ void data_copy_kernel(__gm__ uint8_t* src0Gm, __gm__ uint8_t* src1Gm, __gm__ uint8_t* dstGm) { AscendC::KernelDataCopy op; op.Init(src0Gm, src1Gm, dstGm); op.Process(); }
结果示例:输入数据(src0Global): [1 2 3 ... 512] 输入数据(src1Global): [1 2 3 ... 512] 输出数据(dstGlobal):[2 4 6 ... 1024]
- 随路格式转换数据搬运,nz2nd
#include "kernel_operator.h" namespace AscendC { class KernelDataCopyUb2GmNz2Nd { public: __aicore__ inline KernelDataCopyUb2GmNz2Nd() {} __aicore__ inline void Init(__gm__ uint8_t* dstGm, __gm__ uint8_t* srcGm) { Nz2NdParamsFull intriParamsIn{1, 32, 32, 1, 32, 32, 1}; intriParams = intriParamsIn; srcGlobal.SetGlobalBuffer((__gm__ half *)srcGm); dstGlobal.SetGlobalBuffer((__gm__ half *)dstGm); pipe.InitBuffer(inQueueSrcVecIn, 1, intriParams.nValue * intriParams.dValue * sizeof(half)); pipe.InitBuffer(inQueueSrcVecOut, 1, intriParams.nValue * intriParams.dValue * sizeof(half)); } __aicore__ inline void Process() { CopyIn(); Compute(); CopyOut(); } private: __aicore__ inline void CopyIn() { LocalTensor<half> srcLocal = inQueueSrcVecIn.AllocTensor<half>(); DataCopy(srcLocal, srcGlobal, intriParams.nValue * intriParams.dValue); inQueueSrcVecIn.EnQue(srcLocal); } __aicore__ inline void Compute() { LocalTensor<half> dstLocal = inQueueSrcVecIn.DeQue<half>(); LocalTensor<half> srcOutLocal = inQueueSrcVecOut.AllocTensor<half>(); DataCopy(srcOutLocal, dstLocal, intriParams.nValue * intriParams.dValue); inQueueSrcVecOut.EnQue(srcOutLocal); inQueueSrcVecIn.FreeTensor(dstLocal); } __aicore__ inline void CopyOut() { LocalTensor<half> srcOutLocalDe = inQueueSrcVecOut.DeQue<half>(); DataCopy(dstGlobal, srcOutLocalDe, intriParams); inQueueSrcVecOut.FreeTensor(srcOutLocalDe); } private: TPipe pipe; TQue<QuePosition::VECIN, 1> inQueueSrcVecIn; TQue<QuePosition::VECOUT, 1> inQueueSrcVecOut; GlobalTensor<half> srcGlobal; GlobalTensor<half> dstGlobal; Nz2NdParamsFull intriParams; }; } // namespace AscendC extern "C" __global__ __aicore__ void kernel_data_copy_nz2nd_ub2out(__gm__ uint8_t* src_gm, __gm__ uint8_t* dst_gm) { AscendC::KernelDataCopyUb2GmNz2Nd op; op.Init(dst_gm, src_gm); op.Process(); }
结果示例:输入数据(srcGlobal): [1 2 3 ... 1024] 输出数据(dstGlobal):[1 2 ... 15 16 513 514 ... 527 528 17 18 ... 31 32 529 530 ... 543 544 ...497 498 ... 511 512 1009 1010... 1023 1024]
- 切片数据搬运,非连续转为连续
#include "kernel_operator.h" using namespace AscendC; // 本样例中tensor数据类型为float template <typename T> class KernelDataCopySliceGM2UB { public: __aicore__ inline KernelDataCopySliceGM2UB() {} __aicore__ inline void Init(__gm__ uint8_t* dstGm, __gm__ uint8_t* srcGm) { SliceInfo srcSliceInfoIn[] = {{16, 70, 7, 3, 87}, {0, 2, 1, 1, 3}};// 如输入数据示例:startIndex为16,endIndex为70,burstLen为3,stride为7, shapeValue为87。 SliceInfo dstSliceInfoIn[] = {{0, 47, 0, 3, 48}, {0, 1, 0, 1, 2}};// UB空间相对紧张,建议设置stride为0。 uint32_t dimValueIn = 2; uint32_t dstDataSize = 96; uint32_t srcDataSize = 261; dimValue = dimValueIn; for (uint32_t i = 0; i < dimValueIn; i++) { srcSliceInfo[i].startIndex = srcSliceInfoIn[i].startIndex; srcSliceInfo[i].endIndex = srcSliceInfoIn[i].endIndex; srcSliceInfo[i].stride = srcSliceInfoIn[i].stride; srcSliceInfo[i].burstLen = srcSliceInfoIn[i].burstLen; srcSliceInfo[i].shapeValue = srcSliceInfoIn[i].shapeValue; dstSliceInfo[i].startIndex = dstSliceInfoIn[i].startIndex; dstSliceInfo[i].endIndex = dstSliceInfoIn[i].endIndex; dstSliceInfo[i].stride = dstSliceInfoIn[i].stride; dstSliceInfo[i].burstLen = dstSliceInfoIn[i].burstLen; dstSliceInfo[i].shapeValue = dstSliceInfoIn[i].shapeValue; } srcGlobal.SetGlobalBuffer((__gm__ T *)srcGm); dstGlobal.SetGlobalBuffer((__gm__ T *)dstGm); pipe.InitBuffer(inQueueSrcVecIn, 1, dstDataSize * sizeof(T)); } __aicore__ inline void Process() { CopyIn(); CopyOut(); } private: __aicore__ inline void CopyIn() { LocalTensor<T> srcLocal = inQueueSrcVecIn.AllocTensor<T>(); DataCopy(srcLocal, srcGlobal, dstSliceInfo, srcSliceInfo, dimValue); inQueueSrcVecIn.EnQue(srcLocal); } __aicore__ inline void CopyOut() { LocalTensor<T> srcOutLocal = inQueueSrcVecIn.DeQue<T>(); DataCopy(dstGlobal, srcOutLocal, dstSliceInfo, dstSliceInfo, dimValue); inQueueSrcVecIn.FreeTensor(srcOutLocal); } private: TPipe pipe; TQue<QuePosition::VECIN, 1> inQueueSrcVecIn; GlobalTensor<T> srcGlobal; GlobalTensor<T> dstGlobal; SliceInfo dstSliceInfo[K_MAX_DIM]; SliceInfo srcSliceInfo[K_MAX_DIM]; // K_MAX_DIM = 8 uint32_t dimValue; }; extern "C" __global__ __aicore__ void kernel_data_copy_slice_out2ub(__gm__ uint8_t* src_gm, __gm__ uint8_t* dst_gm) { AscendC::KernelDataCopySliceGM2UB op; op.Init(dst_gm, src_gm); op.Process(); }
结果示例:
输入数据(srcGlobal):
00000000
00000000
11111111
11111111
11111111
0000000(7个0)
11111111
11111111
11111111
00000000
00000000
00000000
00000000
00000000
00000000
00000000
0000000(7个0)
00000000
00000000
00000000
00000000
00000000
00000000
00000000
11111111
11111111
11111111
0000000(7个0)
11111111
11111111
11111111
00000000
00000000
输出数据(dstGlobal):
11111111
11111111
11111111
11111111
11111111
11111111
11111111
11111111
11111111
11111111
11111111
11111111